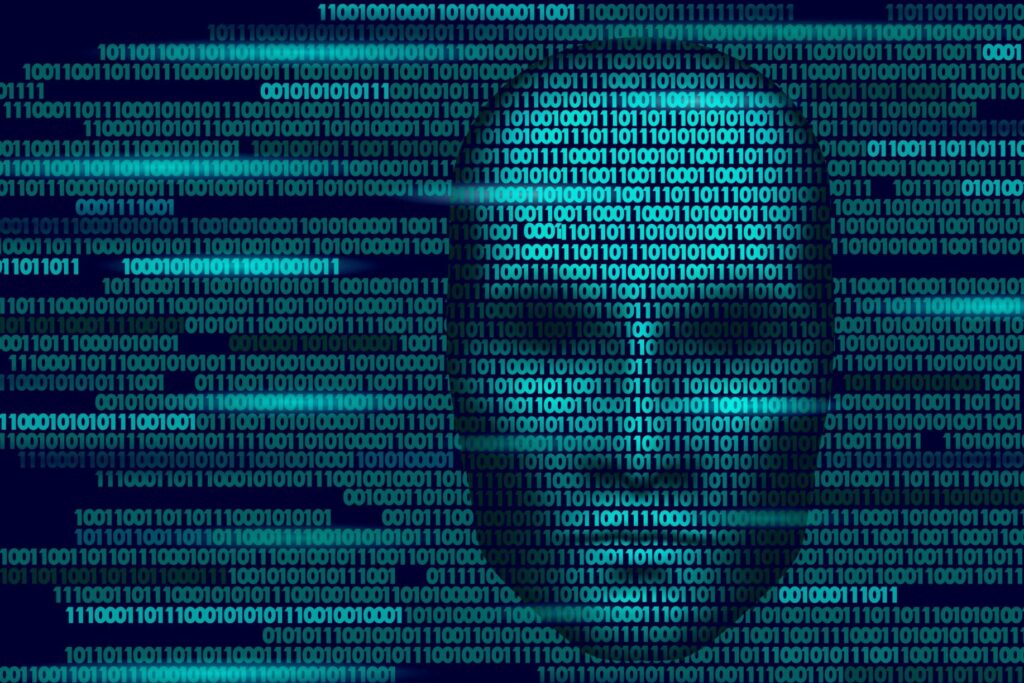
Artificial intelligence (AI) has rapidly emerged as a transformative force in various industries, reshaping how businesses operate, make decisions, and engage with customers. While the rapid advancement of AI technology brings remarkable opportunities, it simultaneously introduces complex challenges that require fresh perspectives. These challenges encompass trust, risk, and security management and extend far beyond the capabilities of conventional controls.
Traditional controls and frameworks are ill-equipped to address these novel challenges, so data and analytics leaders (D&A) must be prepared to alter their operating models to improve reliability, trustworthiness, fairness, privacy, and security.
Safe and Effective Implementation of AI TRiSM
Organisations face a dual mandate of harnessing AI’s potential while adhering to ethical and regulatory requirements. The imperative for AI to be transparent, reliable, fair, and secure has never been more critical. Regulatory bodies and stakeholders now demand responsible AI practices that safeguard against bias, discrimination, and unintended consequences. The AI Trust, Risk, and Security Management (AI TRiSM) is a framework designed to equip data and analytics leaders with the essential capabilities needed to enhance model reliability, trustworthiness, fairness, privacy, and security. It serves as the compass guiding organisations toward responsible and ethical AI deployment.
One of the most significant shifts in managing AI revolves around the approach to security. Traditional security measures are inadequate for the unique characteristics of AI models. Look to adapt to this new reality by reimagining the approach to AI security. Instead of treating AI models as traditional applications, focus on evaluating specific threat vectors, particularly those relevant to enterprise AI applications and third-party applications integrated with AI models. A dedicated AI application security programme is essential, emphasising adversarial attack resistance to safeguard AI models from malicious attacks.
Organisations prioritising these aspects are poised to achieve remarkable improvements in adoption, business goals, and user acceptance by 2026, highlighting the critical importance of integrating AI TRiSM into organisational strategies.
Moreover, the trajectory of the AI TRiSM market points toward an evolution characterised by acquisitions of AI risk management functionality by enterprise risk management vendors. This expansion of capabilities reflects the growing recognition of the central role that AI TRiSM plays in safeguarding AI implementations. Simultaneously, regulatory measures are anticipated to become more stringent, potentially leading to AI deployment bans for noncompliance with data protection or AI governance legislation by 2027.
Distinct Pillars Form the Foundation of Effective Management in the AI Era
Within the AI TRiSM framework, distinct pillars form the foundation of effective management in the AI era. Explainability and model monitoring go beyond accurate predictions, aiming to demystify how AI models function, identify biases, and ensure transparency in decision-making processes. Privacy measures are paramount in the age of AI, where vast amounts of sensitive data are processed. Synthetic data, homomorphic encryption, and secure multiparty computing are emerging as critical safeguards to protect privacy while harnessing the power of AI. Model operations are crucial for end-to-end governance and lifecycle management of AI models, bridging the gap between model development and operationalisation. Lastly, AI application security plays a pivotal role in protecting AI models from malicious attacks, with a focus on adversarial attack resistance, proactive security measures, and continuous monitoring to detect and mitigate threats.
The future trajectory of AI TRiSM unfolds through five distinct phases, each contributing to its maturation and integration into broader AI engineering and governance disciplines. These phases encompass model lifecycle scope expansion, feature collision resolution, model management and feature convergence, market consolidation with expanded capabilities, and AI-augmented TRiSM integration into broader AI governance practices.
The AI landscape introduces unprecedented challenges that demand specialised approaches to trust, risk, and security management. Organisations that embrace the pillars of AI TRiSM are undoubtedly better equipped to ensure the reliability, transparency, and compliance of their AI implementations. By understanding the evolving landscape and adopting effective strategies, IT leaders can guide their organisations toward responsible, ethical, and secure AI deployment.
Gartner analysts will explore how IT and security and risk management leaders must structure their AI operating models at the Gartner Security & Risk Management Summit, taking place from 26 – 28 September 2023 in London.
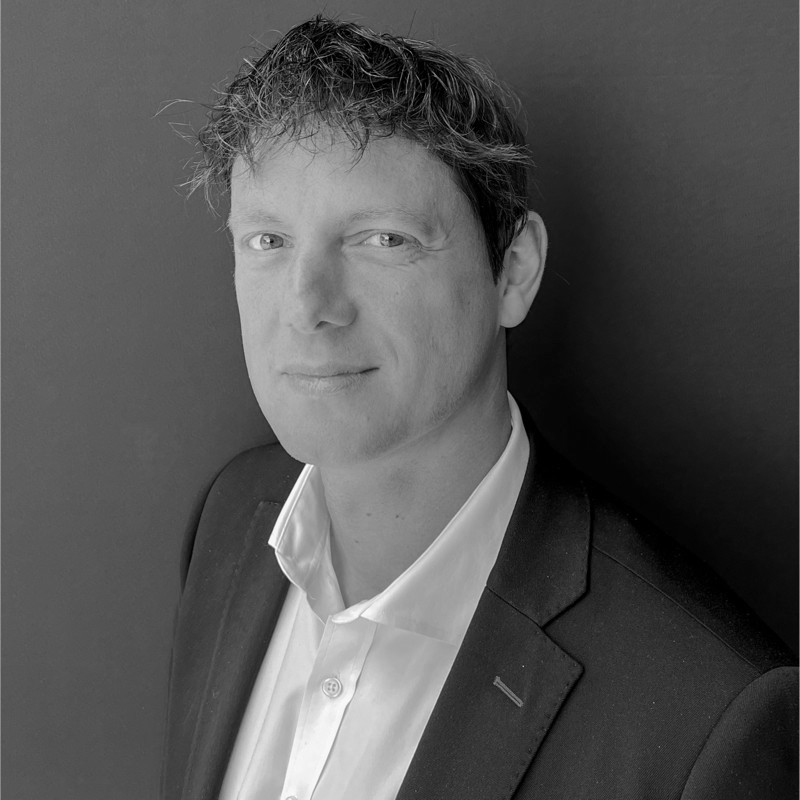
Jeremy D’Hoinne is VP Analyst Gartner.
As a Research VP for security operations and infrastructure protection, Jeremy assists Chief Information Security Officers (CISO’s) and their teams to develop strategies to protect against advanced threats.
Jeremy’s research includes exposure management and how to run a continuous threat exposure management (CTEM) programme, but also how covers the related technologies, such as cybersecurity validation technologies, including breach and attack simulation (BAS). He also studies the intersection of artificial intelligence and cybersecurity with a focus on the disruptions caused by large language models and generative AI.
Jeremy continues to advise organisations on infrastructure protection, especially network detection and response, remote access, and network and micro-segmentation.