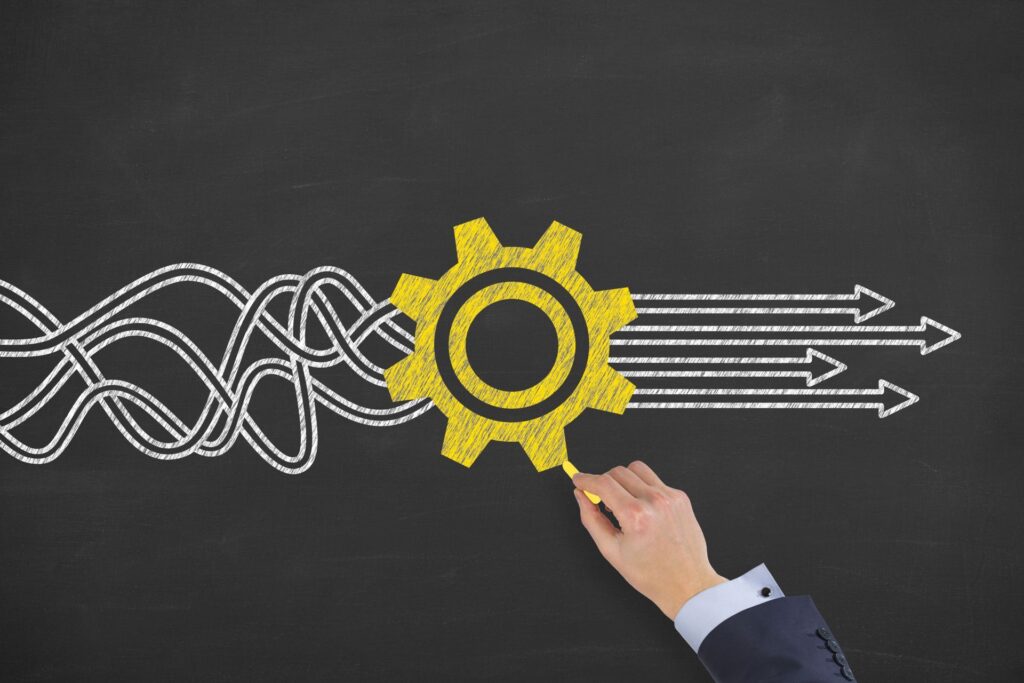
We live in a world where the need to continuously predict what will happen in the future has become table stakes for business survival.
The traditional approaches to actionable predictive insight cannot keep pace with the speed of business. There needs to be an alternative to asking IT teams to pull data from multiple different systems, manually joining, aggregating and cleansing, handing off to the organisation’s data scientists, having them do manual feature engineering, coding models by hand, and deploying them in an ad hoc fashion.
The need for change is becoming increasingly business critical because it is estimated that experts spend up to 80% of their efforts on finding, cleaning and shaping the data, rather than getting the real insights into business problems they need to solve. Clearly there are a number of challenges that need to be overcome.
Firstly, the individuals who have the ability to do the data preparation work are not the same individuals as those who have the business context for the analytic task at hand. Secondly, the tools designed for doing this work were built during a time where the primary enterprise data was relational data in databases. Third is that each piece of the data preparation lifecycle, from ingesting, to profiling, to transforming, to cleansing, to delivery is housed in disparate tools that were never designed to work together. It’s also important to note that the tools that were designed for data preparation have been completely manual, requiring individuals to do the work one step at a time with little machine assistance.
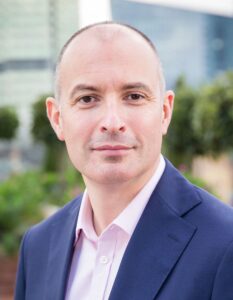
The huge volume and variety of data that can now be collected has not been matched by an increase in the number of data scientists who are available to implement machine learning initiatives and drive advanced analytics. The shortage of data scientists means that organisations need to find a more efficient way to turn raw data into usable information. The focus needs to be on predictions that drive actions aligned with optimising business objectives, not basic data preparation.
What are the cornerstones to enterprise-grade data prep that could offer a solution to this conundrum?
Of utmost importance is a user-centric design approach that puts the capabilities of data preparation into the hands of data scientists and business analysts. A familiar visual approach, high degree of interactivity, and rich library of transformations that resemble tools like Microsoft Excel can create a much more comfortable environment for non-ETL developers to prepare data. The ability to automatically ingest and varse hugely diverse data – from structured data from relational databases, file based sources including modern data lakes like Amazon S3, Azure ADLS, and Google Cloud Storage, modern semi-structured formats such as JSON and XML used to power web applications, to unstructured data including logs, images and geospatial information – is also imperative.
Similarly, the ease with which data can be profiled and data quality issues can be proactively identified is also key. Simple calculations like type conformance are valuable, but modern technologies can go way beyond this and even identify those columns that need to be standardised by identifying the slight variations in spelling of words.
Modern data preparation can also provide a rich set of fundamental data transformation operations that can be applied on large datasets with very fast response times. This means that users could immediately see the impact of their transformations and understand whether they are correct or not.
Enterprise-grade data prep should have native machine learning built into the platform for common data preparation tasks. Modern tools can automatically determine how to join disparate data sets, normalise them, and automate their processing with very little human intervention. Even further, for machine learning use cases, automated feature discovery can analyse the data and generate new features such as ratios and differences of existing variables in the data to determine if they improve the prediction accuracy of a model.
Enterprise governance capabilities will also be critical. Being able to see the lineage across an entire set of data flows, tracing individual columns throughout their lifecycle and even individual values, is crucial in providing transparency. The system should also provide the ability to version all of the datasets and transformation for complete reproducibility, which is so critical with ML pipelines, for example.
Finally, it should be a given that collaboration is a fundamental part of the enterprise data preparation process. Allowing people to share data flows, data sets, tag data, and add annotations allows for a seamless human-centred approach to data preparation expected of a user community that has grown accustomed to having these capabilities at their disposal.
The combination of data scientist-centric, machine-learning powered, interactive, and collaboratively governed data preparation capabilities can dramatically reduce the amount of time users spend in the data preparation process, freeing them up to focus on what really matters, which is extracting business value from analytic capabilities.
Working alongside other modern automated technologies, such as automated machine learning and automated deployment, enterprise data preparation can dramatically increase the velocity of iteration of machine learning workloads. In a world where speed of response can separate winners from losers, enterprise-grade data prep could be the answer businesses are looking for.
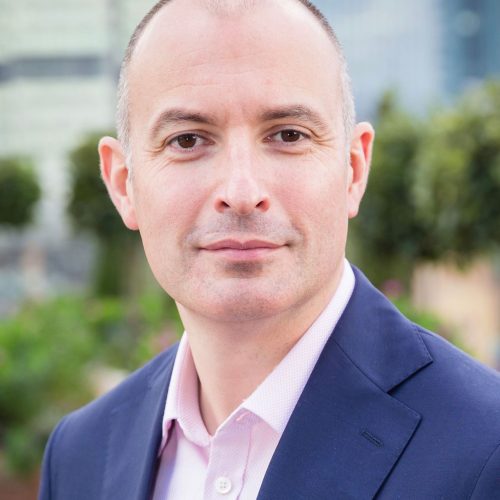
Simon Blunn
VP & GM EMEA, DataRobot