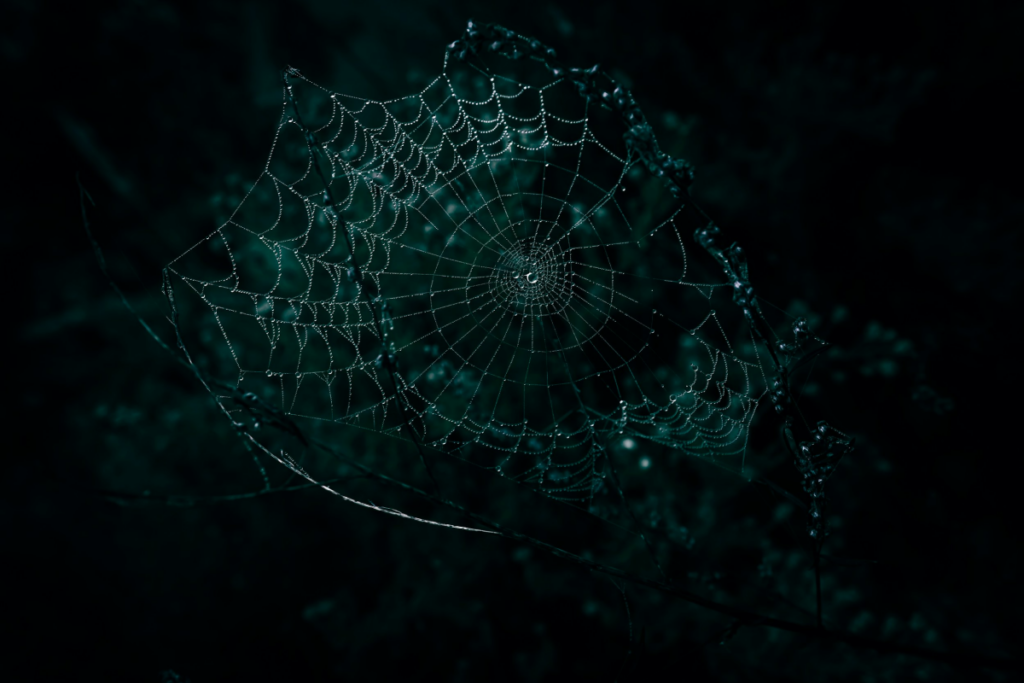
Artificial Intelligence (AI) promises to enhance Enterprise Resource Planning (ERP) systems by offering automation, predictive analytics, and real-time insights. However, there’s a growing recognition that the overuse or misapplication of AI within ERP systems can lead to unintended consequences. This paradox, which I’m calling “The AI Trap,” represents the delicate balance between innovating for the future and avoiding costly overreach.
ERP vendors are investing heavily in AI and generative AI innovation, providing tools that can support companies in navigating these challenges. Solutions such as AI-driven data analytics, automated workflows, and intelligent decision-making platforms are becoming increasingly sophisticated and industry specific. Vendors also offer consulting services to ensure that companies choose the most appropriate use cases for AI and adopt solutions that match their specific needs.
While AI is considered an essential ERP capability, its implementation, can create complications if not approached with a thorough understanding of an organisation’s specific requirements. A new EY AI Pulse Survey shows that the number of senior leaders investing $10m or more in AI is expected to double in 2025, but that many are failing to invest in the necessary infrastructure.
The Public Sector
A notable example comes from the public sector, where AI-driven ERP systems have been touted as the solution to streamline government operations. However, many of these systems struggle to align with the complex, unique requirements of government agencies. In one case, a state government implemented an AI-powered ERP system designed for commercial enterprises, hoping it would modernise its financial reporting and operations.
Unfortunately, the system’s lack of support for government-specific needs, such as fund accounting and complex budget controls, led to significant inefficiencies. Workarounds and “shadow systems” emerged as employees reverted to old methods to get their work done, ultimately leading to the abandonment of the AI-driven solution. This not only resulted in wasted resources but also necessitated a costly return to a more traditional, purpose-built ERP system. (Nurski, L. (2023) ‘AI adoption in the public sector: a case study’, Working Paper 03/2023, Bruegel).
As few real case examples of AI failures relating specifically to ERP projects have been published, let’s consider some other industry-specific scenarios where over-reliance on or unpreparedness for AI could lead to costly setbacks.
Nonprofits
A major global nonprofit implements AI to enhance donor relationship management within its ERP system. The AI tool is designed to analyse donor behaviour, predict future contributions, and optimise outreach campaigns. Despite guidance from an AI vendor, the organisation encounters unforeseen problems: algorithms prioritise high-value donors, ignoring smaller contributors, and impersonal communications are generated, alienating key supporters.
As a result, the NGO experiences a drop in donations, forcing the nonprofit to scale back the AI system and reintroduce manual processes to repair relationships. In this scenario, vendor support couldn’t entirely prevent AI from disrupting the nonprofit’s human-centred approach. This underscores the importance of balancing technological innovation with the organisation’s core values.
Professional Services
In the professional services industry, a mid-sized consulting firm decides to integrate AI into its ERP system to improve project management and resource allocation. Initially, the AI algorithms provide insights that seem promising, such as predicting project overruns and optimising staff assignments. However, the firm’s unique business model, which requires a high degree of flexibility and human judgment, clashes with the AI’s standardised approach. Over time, the firm notices that the AI-driven decisions are leading to inefficiencies, such as assigning the wrong personnel to critical projects, ultimately resulting in client dissatisfaction. The firm then has to roll back the AI components, reintroducing human oversight and discretion into the process—a costly and time-consuming endeavour.
Balancing Innovation with Practicality
Cloud platforms and AI are revolutionising ERP systems by offering immediate data access, sophisticated analytics, and more efficient operations. To fully leverage the benefits of AI, businesses need to invest in robust cloud infrastructure and a comprehensive data strategy.
Effective data governance is critical to ensuring the ethical and compliant use of AI in ERP systems. Companies should establish a comprehensive AI framework that outlines guiding principles for responsible AI development and deployment. An AI policy is essential to define the processes staff must follow to ensure AI tools are used ethically and in compliance with legal requirements. Additionally, organizations should implement a product development review process to ensure that any AI integrated into their products adheres to these guiding principles.
The scenarios highlighted above underline a critical paradox in the deployment of AI within ERP systems: while AI can drive innovation and efficiency, its overuse or forced integration can lead to significant setbacks. Organizations must carefully assess whether AI is the right tool for their specific needs and remain cautious of the allure of technology for technology’s sake. Balancing the promise of AI with the practical realities of an organization’s operations is crucial to avoid the costly pitfalls of misaligned AI integration.
Human Centric-AI Empowering Human Potential
At Unit4, we do not believe AI, particularly GenAI, is applicable in every scenario. Automation can drive a lot of productivity and efficiency improvements, but unless it is focused on solving real problems that users are facing, it lacks purpose.
We believe the best approach, which we recommend to customers, is defining the problem first. What question are you looking to answer? If you know this, then you can define what data you need to address the question. This is also important, because the temptation with AI is to believe that all data is good (and relevant) to an AI system. In reality, some questions can be resolved more efficiently using small data models rather than trying to crunch vast amounts of data in large language models.
With the right support, organizations can realize AI’s full potential without falling into the pitfalls of over-automation or under-preparation.
Prepare now for upcoming advancements from ERP vendors by examining your existing processes and workflows, identifying repetitive tasks, and being ready to implement new functionalities as they become available.
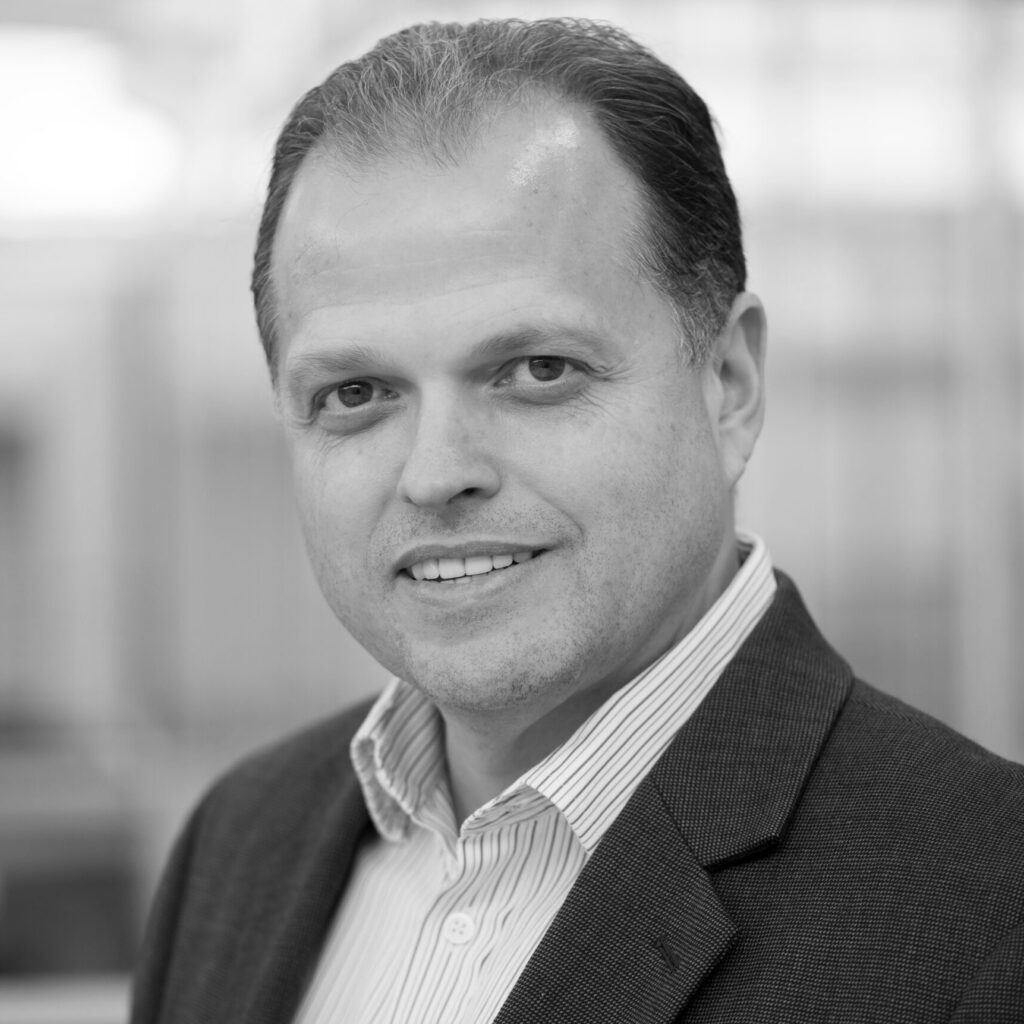
Mike Ettling
Mike Ettling is a respected leader in IT, HCM, and cloud software. Currently the CEO of Unit4, he champions a people-centric vision, believing in the transformative power of enterprise technologies. Previously, he served as President of SAP SuccessFactors and CEO of NGA HR. He has also held key positions at Unisys. Mike holds a master’s degree in Finance from London Business School and is a graduate of the University of Witwatersrand, South Africa.