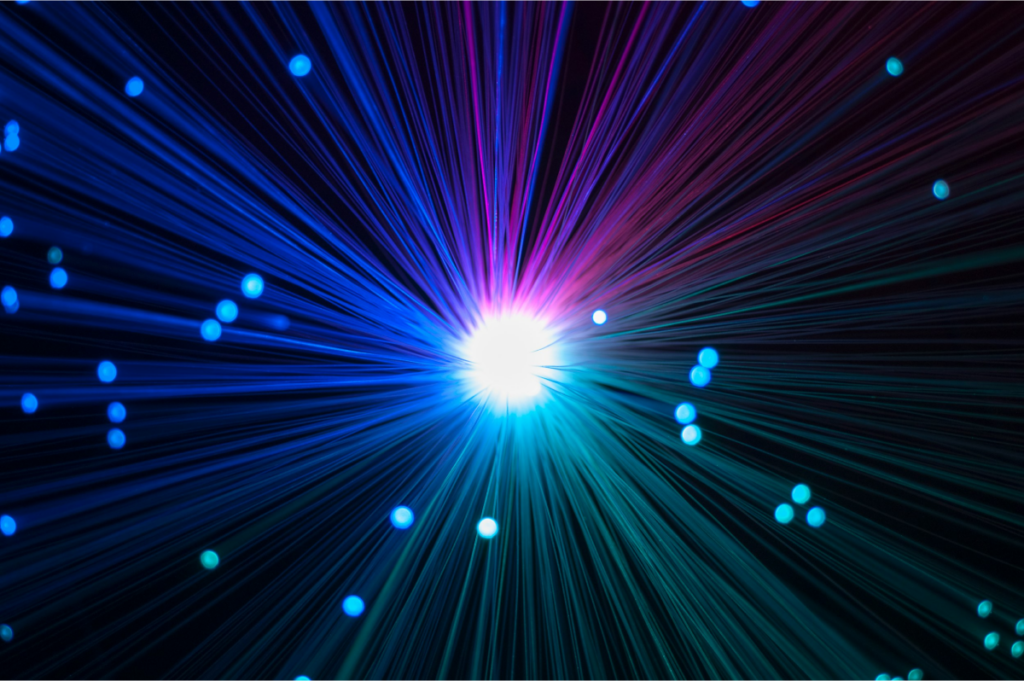
The transformative potential of artificial intelligence (AI) is putting organisations under pressure to implement new tools and systems at speed. The challenge is exacerbated by demands from the C-Suite, who are eager to harness the benefits of AI. Recent studies highlight this urgency, with Gartner reporting that a majority of top executives—62% of CFOs and 58% of CEOs—anticipate AI will profoundly shape their industries within the next three years.
Technical teams, acutely aware of the complexities involved, recognise that hasty implementation can be counterproductive. This drive for rapid deployment creates an opportunity to align business momentum with technical excellence. The question then becomes: How can organisations strike a balance between the C-suite’s demand for swift AI adoption and the technical team’s need for a methodical, charted approach?
Strategic considerations for AI deployment
To fully grasp the opportunity at hand, it’s crucial to understand the key considerations for successful AI implementation through a well-defined roadmap. A primary concern is over-implementation, where multiple tools with overlapping functionalities are deployed simultaneously. This scenario often results in redundant efforts, squandering valuable resources and incurring unnecessary expenses. While the enthusiasm driving rapid adoption demonstrates promising innovation potential, targeted and strategic approaches typically yield the best results.
The advent of generative AI has highlighted the strategic value of high-quality data and information management. The accuracy of AI model outputs directly influences business decisions, making data quality a key success factor. . Consider a scenario where an employee relies on AI to determine customer offers: if the model produces an incorrect recommendation that is then implemented, it could significantly impact the company’s financial performance. Additionally, if incorrect information is provided and supplied to stakeholders, this risks damaging the organisation’s reputation and eroding trust. With over half of AI users already reporting difficulties in achieving desired outcomes from AI systems, the stakes in a business context are exponentially higher.
While data quality is paramount, the selection and implementation of AI models are equally crucial. This is particularly true for heavily regulated industries, though it remains a significant consideration across all sectors. Organisations can enhance their decision-making processes by ensuring model outputs are reproducible and traceable. This creates an opportunity to establish models that are reliable, consistent, and secure. The quality of data used in model training is of utmost importance—it serves as the lifeblood of AI, directly influencing the accuracy and trustworthiness of the model’s outputs.
Rushing the implementation risks overlooking key steps, such as ensuring the use of high-quality, accurate data. A structured implementation approach, incorporating thorough data quality verification, helps organisations build sustainable AI systems. . While technical teams bring valuable expertise to these considerations, developing clear communication channels with the C-suite can help align technical and strategic priorities.
The power of controlled AI experimentation
In the face of these challenges, a promising middle ground emerges: controlled AI experimentation. This approach offers a viable solution that can satisfy both the C-suite’s desire for progress and the technical team’s need for robust development. Recent MIT research reveals a significant trend, with 56% engaging in experimental model logging compared to the previous year and rightly so. By focusing on addressing specific business ‘pain points’, this approach can effectively bridge the gap between business objectives and technological implementation.
Controlled experimentation with generative AI offers multiple advantages. Firstly, it aids in identifying the most impactful use cases for an organisation. By deploying AI in a safe, controlled environment, issues can be detected and resolved proactively. For example, if a model provides inaccurate responses to employees, developers can analyse and refine the training data before the official rollout. This process also helps in determining necessary governance structures, such as establishing operating models or coordinated hand-offs between teams to manage the end-to-end generative AI cycle. Further experimentation can reveal both areas of skill abundance and deficiency, in turn enabling organisations to plan for any future upskilling programmes. Perhaps most crucially, experimentation can uncover potential data issues that must be addressed to fully operationalise any generative AI model.
This experimental approach satisfies the requirements of both the C-suite and technical teams. From the C-suite’s perspective, it demonstrates tangible progress in AI implementation, alleviating concerns about falling behind competitors. Simultaneously, it affords technical teams greater control over the pace and quality of the rollout. However, for this experimentation to be truly effective, technical teams must adhere to several key principles.
Technical foundations for successful AI experimentation
A fundamental enabler for generative AI is the consolidation or unification of data sources. Utilising platforms such as a Data Intelligence Platform can centralise both data and models, offering organisations a singular access point for their generative AI use-cases. It is equally important to identify and implement AI tools that provide a secure environment for experimentation, enabling end-users to access and validate various Large Language Models (LLMs) to determine the most suitable options for their specific needs. Lastly, establishing robust governance frameworks is essential. These structures allow organisations to effectively monitor and manage access to data and models, as well as track performance and lineage—all critical components for the successful deployment of generative AI.
In the competitive landscape of AI adoption, striking the right balance between swift implementation and meticulous development is crucial. While the C-suite’s push to deploy AI solutions is justified by potential competitive advantages, technical teams rightly emphasise the risks associated with hasty rollouts. This balanced approach paves the way for AI systems that are not only innovative but also reliable, ethical, and aligned with long-term business objectives.
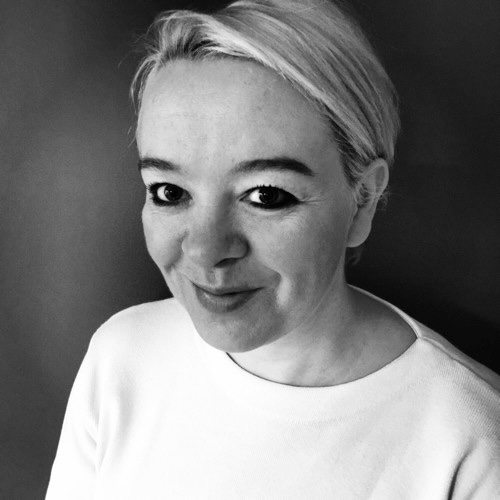
Lynne Bailey
Lynne Bailey is currently Lead Data Strategist at Databricks. She is formerly the Chief Data Officer and People Partner for Technology at KPMG. She held this role for 4 years, and joined KPMG from PwC, where she had spent a large chunk of her career. Lynne has a passion for getting value from data, and using it to enable organisations, and this has been her mission from the start of her career.