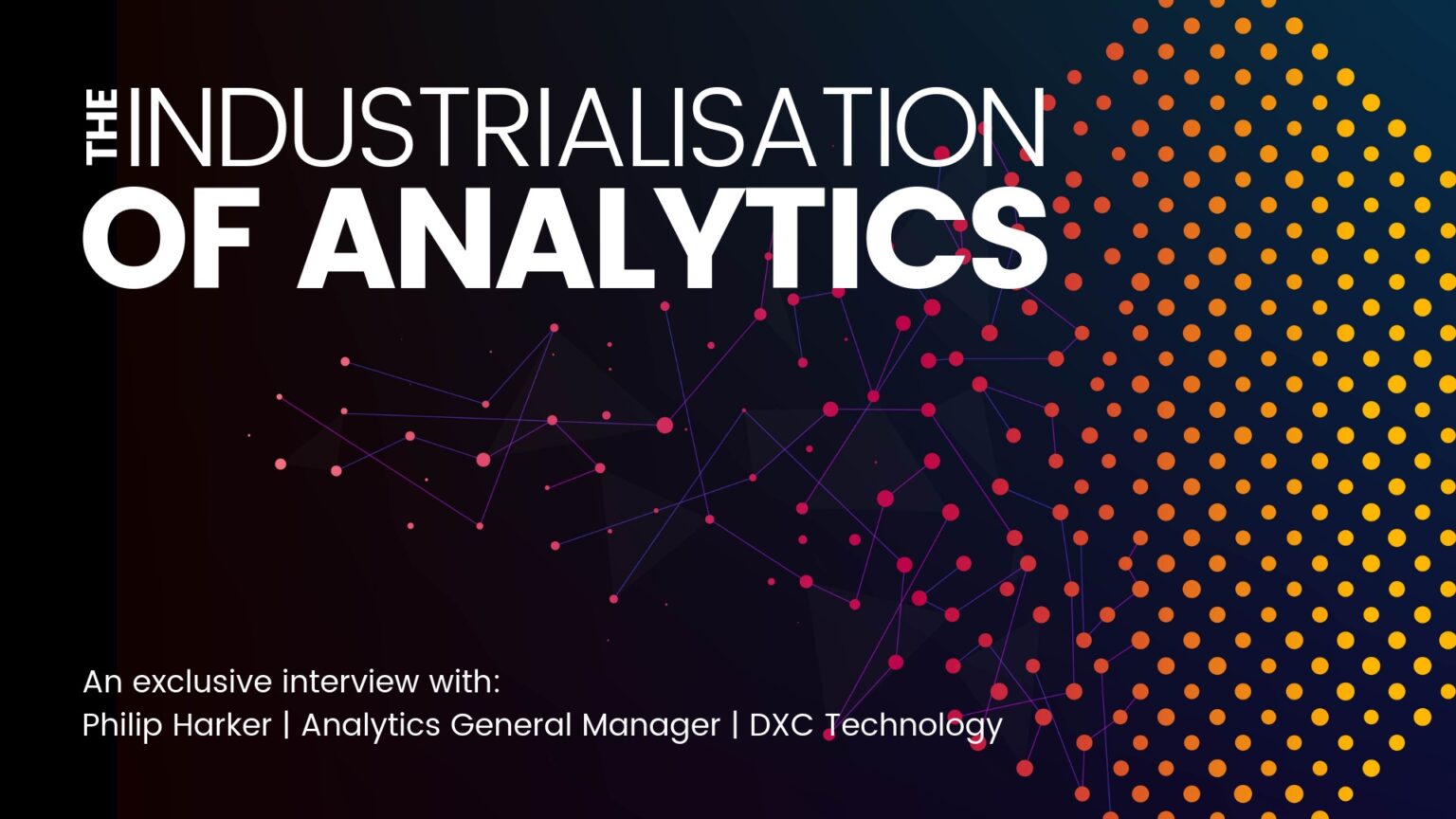
Data crunching isn’t a new challenge in enterprise. Businesses have always pushed to convert hard numbers into meaningful outcomes, though this has traditionally been a labour-intensive and costly task.
Over the past 20 years, technology has edged companies closer to the strategic sweet spot of evidence-based decision making. But as they skilled up on data science and integrated rudimentary analytics tools, a problem emerged: an organisation’s data sets were rapidly outgrowing its capabilities to manage and extract value from them. The term ‘big data’ entered the business lexicon – and technology was forced to play catch-up once again.
Fast-forward to today, and companies – large and small, industrial and consumer – are still striving to gain maximum insights from the mountains of information at their disposal. The race to deliver an all-encompassing solution is hotly-contested and involves some of the world’s major technology players.
Innovation in this area has been based around artificial intelligence (AI) and machine learning – and utilising the newest forms of these technologies is now firmly established as the catalyst for unlocking the potential of data. Philip Harker, Analytics General Manager for DXC Technology, says enterprise is reaching the point of true transformation.
“For many years we have been developing machine learning and AI solutions, initially on exciting use cases like image detection for counterfeit product detection or image analysis in other examples,” Harker explains to Digital Bulletin.
“These innovations have helped us develop the models and learnings. Now is the time to industrialise these machine learning and AI instances and implement them in the overall business process to drive outcomes. For example, at DXC we have developed an AI solution for automating real-time decision processing in services procurement for a financial services customer, enabling the client to become AI-driven.”
Financial services is one of two major sectors where Harker believes the ‘industrialisation’ of AI will have a huge impact on data optimisation, the other being healthcare. The global healthcare analytics market, currently worth $14 billion, will rocket to more than $50 billion in five years, according to recent projections by Markets and Markets.
Machine learning underpins most successful advanced analytics use cases. Algorithms for machine learning have existed for many years but only recently have these complex mathematical calculations been successful in understanding immense volumes of data. Passing this milestone will ultimately benefit both organisations and their customers, according to Harker.
“In finance, for example, AI is used in fraud detection or risk exposure, and to support better governance,” he says. “In healthcare, it is about better patient outcomes through the use of deep analytics to find patterns and to augment data sets. All these uses are made possible by vast amounts of data and compute available on tap, and both these industries are highly regulated.
“It’s all about AI but in reality, when scratching below the surface, many instances are about machine learning. One fine example of this is robotic process automation, or RPA – which is not possible without machine learning to drive it. Often RPA is embedded in a solution as a bot, but the tuning and sharpening of machine learning is critical to enable the value of RPA to be realised.”
Additionally, an emerging challenge is customer demand for instant access to high-value analytics. Providers like DXC are having to find a balance between prioritising client delivery and maintaining innovation in the space, a methodology known as ‘DataOps’. Achieving a streamlined approach to analytics will be key if data optimisation at scale is to sweep across the enterprise.
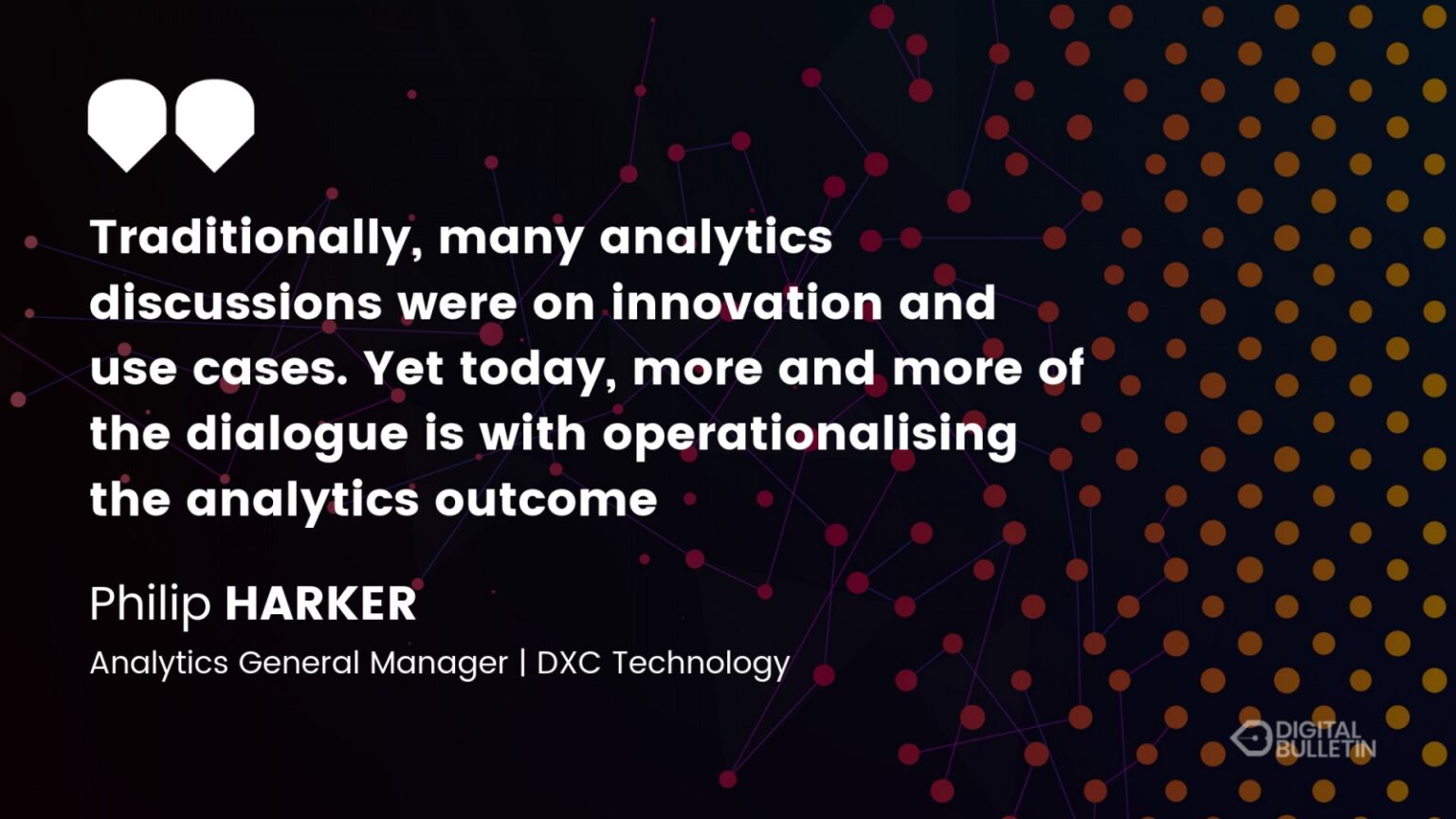
“Traditionally, many analytics discussions were on innovation and use cases,” outlines Harker. “Yet today, more and more of the dialogue is with operationalising the analytics outcome, coupled with the demand for self-service analytics – where users expect data, models and insights at their fingertips to develop their own findings, which in turn can be put back into the repository for general consumption. So, the democratisation of data is the new norm.”
DXC’s own analytics services, which embeds advanced analytics tools like AI with legacy techniques such as Business Intelligence, are wrapped into three main areas.
The company’s ‘Industrialised Analytics Business Solutions’ segment covers customer analytics – predicting customer needs by understanding their behaviour – and industry-tailored solutions. It has made a particular mark in the automotive sector, counting six out of the world’s ten biggest manufacturers as clients.
The ‘Information Governance’ service line includes data governance, information life cycle management and work around GDPR while its third area, ‘Data Engineering and Platform Services’, is DXC’s managed services offering for data processing on leading platforms, including dominant public cloud services like AWS, Azure and Google. Cloud has undoubtedly altered the data analytics landscape along with enterprise transitions to hybrid environments, as Harker outlines.
“The proportion of analytics workloads being served by cloud providers has grown exponentially, and many of them have architectures and tools to serve better distributed computing environments,” he adds.
“AWS, Azure and Google are the de facto standards; we are seeing many big data examples of migration from analytics systems to cloud; moreover, we are seeing many moving to a hybrid approach, in which organisations use a combination of cloud services and on-premise systems.
“We have extensive migration services for such workloads, but it is not just a matter of lifting and shifting the workloads. Often data will have to be cleansed as a prerequisite for the migration of services to off-premise. Hence our adjacent service offering of information governance sitting alongside data engineering and platform services.”
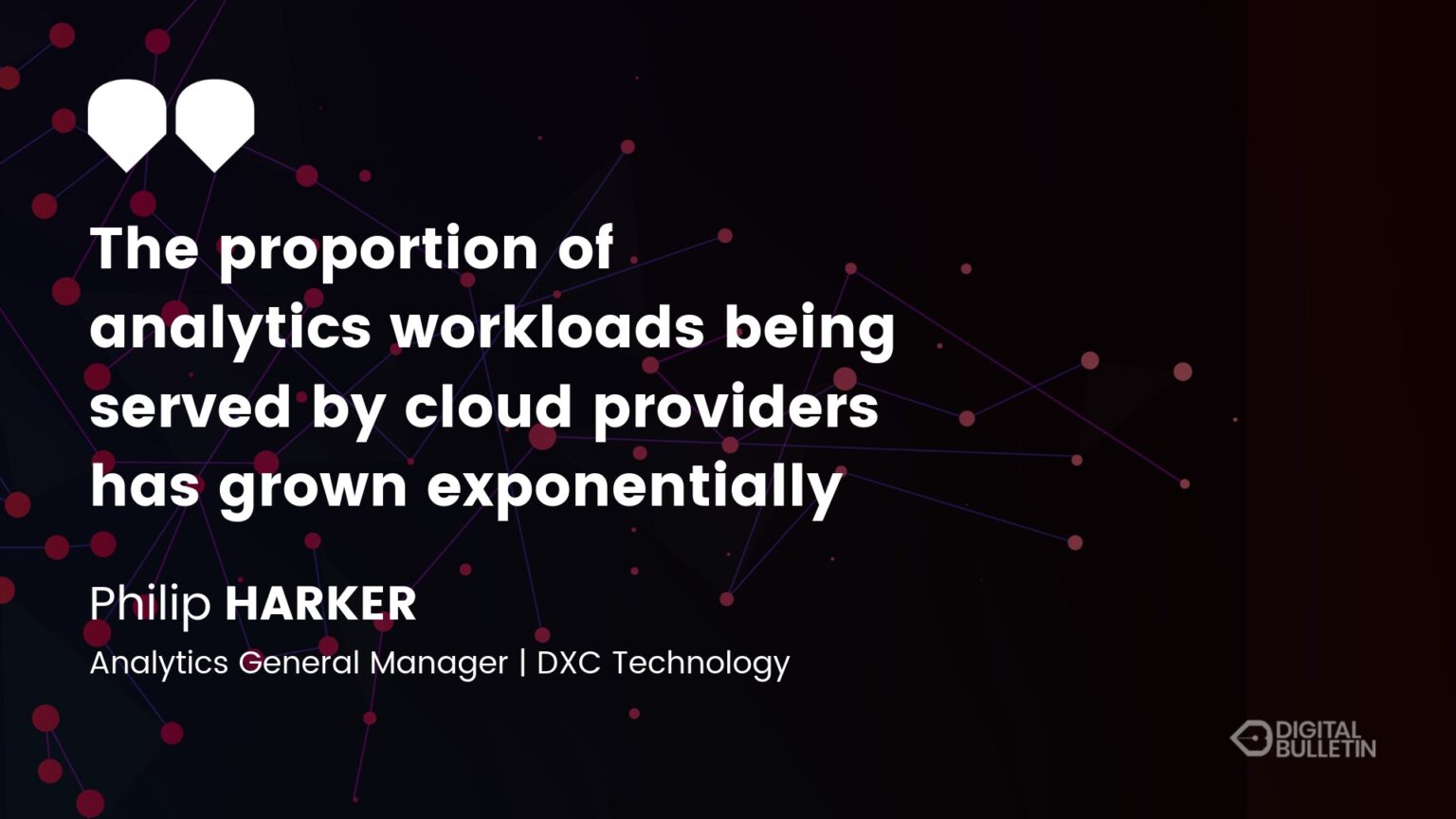
The Internet of Things (IoT) is creating even more opportunities for companies to use data to their advantage – but the unique characteristics of IoT solutions often lead to friction with established data management infrastructures. A Gartner survey of relevant companies found that more than a third would opt for completely separate data management capabilities for IoT.
However, data processing across distributed networks is becoming easier thanks to the growth of open-source software libraries like Apache Hadoop. Harker says DXC is finding a way of capitalising on the potential of IoT and edge computing.
“Distributed computing is not new, but the containerisation on computing code is now becoming the norm, easing the deployment of such environments,” he comments.
“IoT has dramatically matured over recent years, but now it is less about developing use cases and more about edge compute and end-to-end architecture. We are now at the birth of industrialised analytics business solutions around IoT. Our DXC Robotic Drive is a good example. It enables autonomous driving developers to collect, manage and analyse massive amounts of global sensor data at significant speed.
“It not only embraces numerous challenges of distributed compute at scale but also addresses the various characteristics of the data generated by the vehicle. Moreover, the Robotic Drive architecture is the reference platform for productionised IoT applications, a platform that can scale and is based on a consumption model.”
One thing is clear; the challenge of data management has ramped up dramatically as environments and networks grow increasingly complex. Companies are eager to define their data roadmaps but are discovering roadblocks along the way, none more than finding a suitable workforce.
The skills gap is prevalent across the technology sector and is especially stark in data-based specialisms. IBM believes that data science will account for 28% of all technology jobs by the end of 2020 and the European Commission is envisaging 100,000 data-related roles being created in the region within the same timeframe. But is there the supply to meet demand? DXC itself employs more than 8,000 analytics professionals and Harker admits recruitment is at the forefront of its work in the field.
“Recruitment is a constant for us. We have grown our practice head count by 50% over the last year, and we expect this to continue in the future,” he explains. “We have deep expertise in four pivotal domains: data science, data engineering, business consulting and DevOps.
“As is increasingly normal, personally I wear more than one hat. I lead the Solutioning and Advisory for Analytics team in the UK, Ireland, Israel, the Middle East and Africa – a team that sells, advises and architects analytics and data solutions. Secondly, I lead DXC’s major telecom customers in analytics, where I advise and oversee projects and services.
“In both roles, being on topic and staying at the forefront of industry trends is critical for continual developments.”