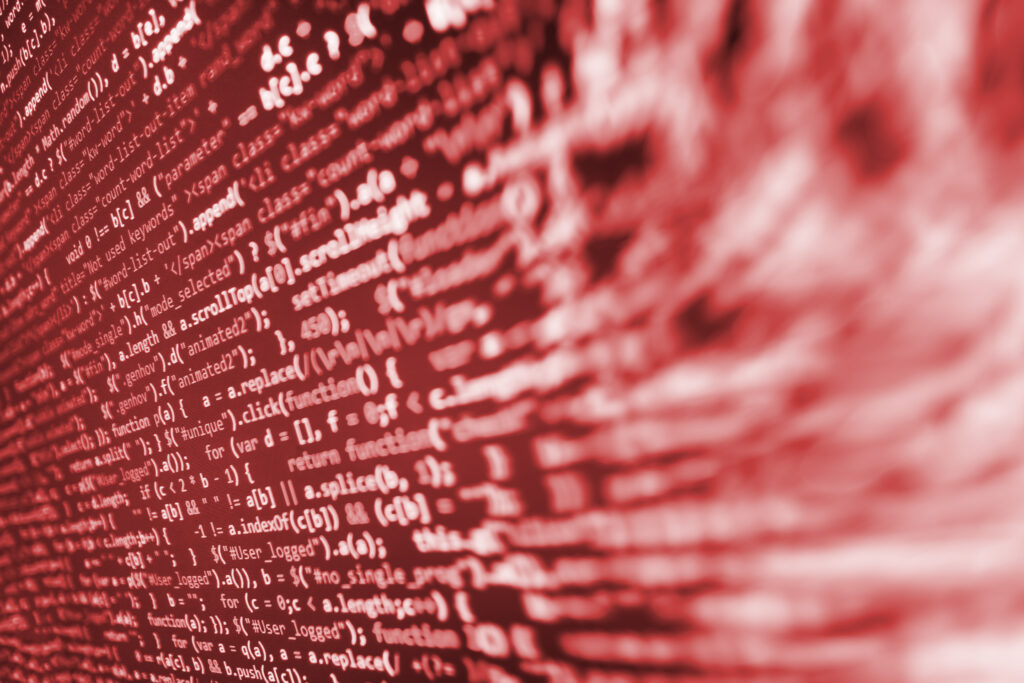
Great data quality equals better insight, improved customer experience, and increased opportunity for innovation. No wonder it is quickly becoming a primary focus for the C-suite. But although a recent HFS Research study found that 95% of executives know their companies would be more competitive and make better decisions if their data was more accurate, the same group say that only 60% of their data is actually usable.
And despite repeated efforts to improve and standardise data, many data projects still end in failure. It is part of my job to spot issues early on and to turn things around before they impact the success of a project. And over the course of more than a decade, I have noticed common themes that, if addressed early on, could help your next data quality initiative hit the mark.
1. Accepting low-quality data as the norm
This is not to say that most enterprises believe low-quality data is a good thing, simply that they may not even realise they have dirty data or – more often – feel that achieving 99+% data quality is impossible.
Setting data quality goals that align with your business KPIs – and tracking the data that matters most to the organisation – really helps here. But having those goals is not enough: the organisations with long-lasting high data quality tend to secure C-suite ownership of those objectives, while making sure that the rest of the organisation understands the goals and is consistently working towards them. Securing buy-in at all levels means that it is not left to the IT team to guarantee data accuracy. It is an organisational responsibility that everyone has a stake in, and this significantly increases success rates.
2. Mistaking expensive analytics or data warehouses for data quality platforms
It can be easy to confuse business analytics platforms, CRMs, and data warehouses for solutions that truly manage and maintain quality data. Although valuable, these sophisticated CRM solutions aren’t developed to address the quality of your data. In fact, even in the case of large-scale data warehouses, the solution is built to house already clean and validated data – something that ideally happens outside the solution.
The complexity and scale at which we now create and use data requires purpose-built software to manage its constant flow throughout the enterprise. Understanding and addressing that early on makes it faster and easier to transform disparate, out-of-date data into a competitive advantage. A side note here though – if you do opt to employ a data management and quality tool, make sure you use it. Too often these valuable solutions end up expensive ‘shelfware’.
3. Not dedicating the right resources to data quality
We have all been there: data quality starts out as a priority, and then gets pushed down the list to become a ‘nice to have’ because the team is stretched or something else becomes more urgent. And it is true, managing a data quality initiative for large-scale, global enterprises can be daunting.
This is where data strategy and governance can be your friend. A collaborative framework that explicitly links to your business goals, to manage and define policies, business rules, and assets, and to provide the necessary level of data quality control. This will make it clear right from the start what level of resource is needed and include touch points to check how your data measures up.
4. Losing buy-in and interest in data quality
We know that data quality is not solely the domain of the IT team. Buy-in from your stakeholders early on is key to driving your data quality initiative across the business. And when I say stakeholders, I mean the rest of your organisation, every single person who creates, manages, and uses data.
Aligning your data quality goals with your business objectives makes it clear why maintaining good data hygiene matters. But this takes time and effort, it is a culture change for many, and needs to be treated as one. Think about how you will bring your organisation along with you, through role modelling, educating, and reinforcing good behaviours.
5. Postponing data quality initiatives until it’s too late
It sounds obvious doesn’t it? Delaying cleaning and updating your data can cost more time, money, and resources in the long run. We all know that dealing with an issue in the early stages will be more straightforward, but data quality often gets pushed down the list of urgency, which is astounding when you consider that analyst firm Gartner found that the average cost of poor data quality on businesses amounts to between $9.7 million and $14.2 million annually.
You will know that the longer you leave data to take care of itself, the knottier the issues become, and the harder it is to tease out what accurate looks like. So it is important to make the case for data quality initiatives early – definitely try not to leave it until you are tackling a migration or transformation. You will have too much on your hands.
Make data quality a priority
Even though most leaders recognise the importance of good quality data, experience tells us that it is still not the operational priority it should be. Understanding why data initiatives fail means you can start to change the way you approach them, and the way they are viewed by the rest of your organisation. These initiatives are not an add on. They are crucial to the success of your business, and the teams that work there. Make that connection, and you will be starting your next data quality initiative ahead of the game
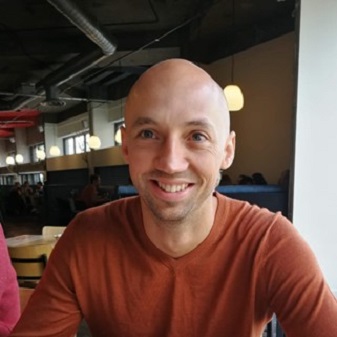
Kevin Wild is responsible for Syniti’s presales function across EMEA. Kevin has over 11 years’ experience supporting complex, global transformations in positions held at Asahi, Coty France, and SABMiller. His experience demonstrates just how critical data is to not just the success of a transformation journey, but also to the success of a company.