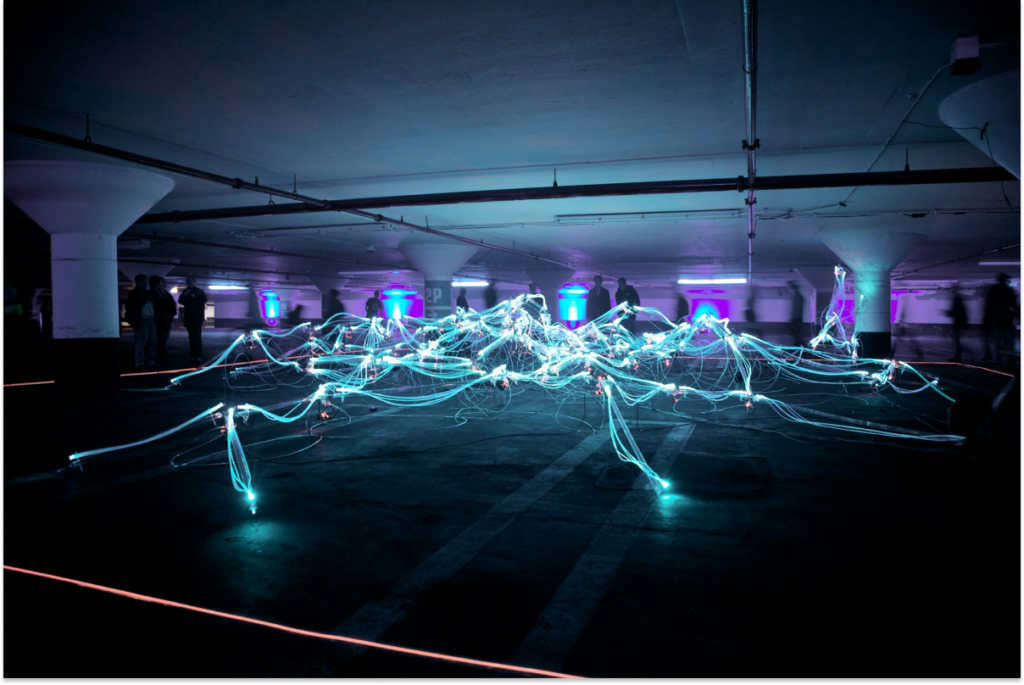
We all recognize the tremendous power and rapid changes being wrought by AI, but we should also pause and recognize that it is amid one of the biggest hype cycles any technology has ever seen. Success in responsible AI planning is therefore as much about setting reasonable expectation parameters for management and non-specialists as it is about deploying the technology itself.
There are already many use cases for AI and related technologies such as Machine Learning. We see wide deployment of smart automation, interactive chatbots and ways to accelerate what were once boring chores. But, even here, the stubborn fact remains that AI deployment today still requires human input, training, careful results validation, security and governance, and significant financial investment. Without factoring in all of these, we will be heading for Gartner’s famous Trough of Disillusionment and AI leaders will be saddled with a reputational challenge similar to those that went too hard and too early into Web 1.0 nearly 30 years ago.
Microsoft, OpenAI, Alphabet, Meta and others have fabulous war chests to throw at technical challenges, but most CIOs are not in the same world of speculative investments in search of moonshots. So, boring as this may sound, conservative analysis of opportunities weighed against risks is the way forward.
To separate sense and nonsense when talking about AI, one practical lens through which to view the challenge is aiming to make every interaction count; that is, to seek tangible positive results for each development program.
Start small
One of the near-term AI opportunities is to augment human tasks and specifically those manual, repetitive tasks that humans tend to hate, and machines tend to be very good at. Spotting errors, generating job descriptions, making expense claims, invoicing, spotting anomalies in payment processing, personalizing user experiences… these are the low-hanging fruit for AI. They may not be the most glamorous use cases, but they work now, and they are exactly the sorts of ‘quick win’ applications that will engender faith and create buy-in to the future of AI. User-centric innovation, pragmatic AI integration and placing ethical responsibility at the front and centre of everything we do are all strong characteristics of where we should think of AI being today. If we get these right, then we can look forward to incremental improvements such as multi-agent interoperability where multiple bots can act in concert.
That’s why pragmatic steps such as the insertion of Generative AI into an ERP extension kit is so important. We can use gen AI to create new workflows and to automate repetitive tasks today: so let’s do that and let’s learn more about prompt optimization and task splitting as we go along.
Our work here has already yielded useful learnings that will inform future investments:
Humans are needed to spot hallucinations. We know that Generative AI can be prone to creating absurd conclusions or appearing to ‘invent’ facts. To increase confidence in AI finding we still need human handholding and sense.
There are a variety of GenAI tools to choose from. Do explore the variety of LLMs available, because all the key players are rushing to move into this space. There are relative merits and costs associated with the different GenAI tools which must be weighed up. It’s wise to avoid lock-in but it requires a thorough evaluation if you want to adopt lesser-known model.
Small Language Models are sometimes useful. Large Language Models have hogged the headlines but small versions can be more affordable and applicable for simple tasks.
Frameworks can help us to focus. Specific models and techniques like Phi-3 or RAG-Fusion may aid us to manage costs and improve precision in generating responses.
Security guardrails need to be everywhere. Quality and security reviews, for example penetration testing exercises, need to be factored in throughout the development process.
Plan to invest. The upfront commitments for incorporating AI shouldn’t be underestimated. You will need to factor in training, infrastructure and process change to develop features.
Think about what success looks like. To maintain focus, it’s important to have a clear strategy about the role of AI in your organization and its likely impact on the profitability of the products and services you offer.
Track high-level governance rules and regs. Knowing about national, international or vertical sector laws, rules and codes is important to the ways in which you design and implement AI, so don’t ignore that changing landscape.
It’s wonderful that we have AI and that it is evolving so quickly. But technologists have a deserved reputation for promising ‘silver bullets’. In this enormous tech wave, it may be politic to err on the side of caution when planning and communicating ways to ride that wave. In so doing we may find that we don’t have to take backward steps or earn the mistrust of colleagues before advancing to the future.
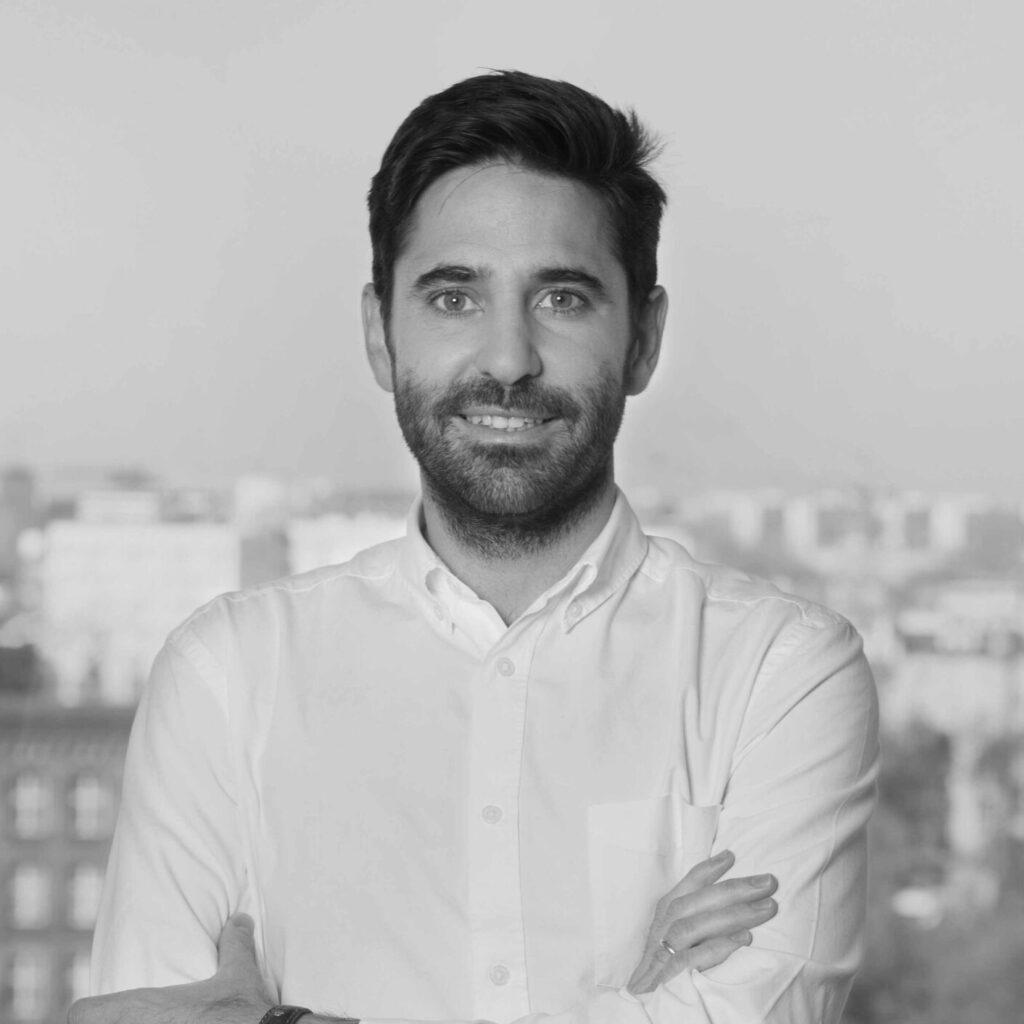
Rafael Artacho
Rafael Artacho is Product Director at Unit4. A seasoned professional in the Software Development sector, Rafael’s 15 year career now sees him playing a key role in the development of the Unit4 People Platform. Rafael has a
keen interest in emerging technologies, particularly Artificial Intelligence, Low-code platforms, Hyperautomation, and cloud-native solutions.