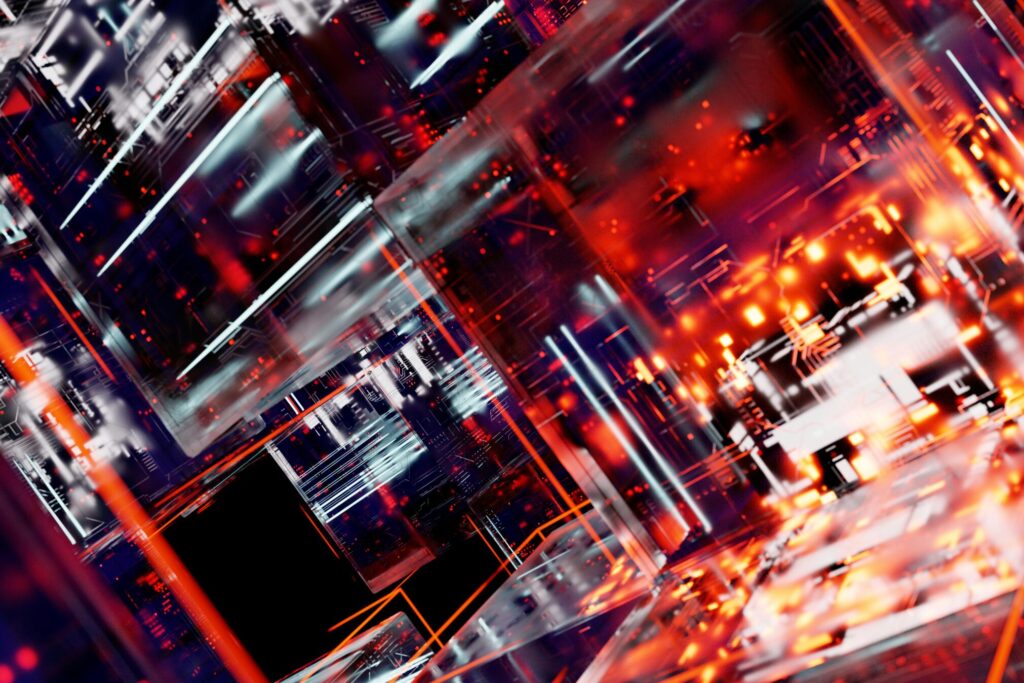
The AI arms race continues to accelerate, with innovation taking place against the backdrop of an emerging regulatory environment. While recent attempts to establish international cooperation, such as the publication of the International AI Safety Report, indicate the potential direction of travel, countries are continuing to push forward individually. In the US, the new administration was quick to announce Project Stargate, a $500 billion private sector initiative supported by OpenAI, Oracle and Softbank, aimed at developing next-generation AI infrastructure. Elsewhere, the UK launched its AI Opportunities Action Plan, backed by £14 billion in tech industry funding.
As is often the case with industries driven by rapid innovation, the gap between progress and regulation is proving difficult to close, at least in the immediate term. Large-scale AI investment in the US was accompanied by the removal of an existing Executive Order designed to guard against the risks AI could present to national security and consumers, including provisions around developmental safety disclosures. Meanwhile, the UK is applying what many describe as lighter-touch governance, with the AI Action Plan focusing on harnessing the potential of the technology and devoting less attention to regulation.
Similarly, proposals to create a National Data Library in the UK that focus on removing “systemic barriers to data access, ensuring that high-quality, AI-ready public-sector data can be safely and efficiently used by scientists and private-sector innovators” raise more security questions than they answer. How, for instance, will the data sets be assembled? Who will take responsibility for data protection? And how can their integrity be guaranteed in the medium and long term when the datasets are used by numerous AI models integral to businesses, public sector services, and the supply chain?
The approach taken by the EU, in contrast, underlines the disparate perspective on AI regulation. Here, the union is adopting a comprehensive, legally binding Act that seeks to regulate AI to ensure transparency and prevent harm. In doing so, it sets out unequivocal obligations for its development and deployment, including mandatory risk assessments and significant fines for non-compliance.
The hidden risks of data poisoning
While the focus on effective regulation is welcome, the continued lack of global coordination increases risk and creates an uneven playing field, where developers in less-regulated countries face fewer implementation barriers than those operating under stricter regimes. In this context, individual organisations have a responsibility to balance innovation with risk mitigation, particularly around cybersecurity in general and the issues associated with data poisoning and the data supply chain in particular.
Take data poisoning, where threat actors deliberately manipulate training data to alter the performance of AI models. This can take various forms, such as introducing minor modifications that generate errors and incorrect outcomes or altering code so it can remain hidden inside a model to retain control over its behaviour. It can occur at various stages in the data lifecycle, from initial stages during data collection to later when injected into data repositories or even introduced inadvertently from other infected sources.
Implementing AI is already a risk-reward balancing act, and the stakes will continue to rise as the technologies are more deeply integrated into tech infrastructure and business processes
Either way, this kind of activity introduces significant risk, whereby organisations that have been compromised over a period of time leave themselves open to poor decision-making and, where relevant, regulatory breaches. Preventing it is dependent on processes such as data validation and anomaly detection, backed by continuous dataset monitoring to identify and remove malicious changes.
Supply chain vulnerabilities need forward planning and resilience
Turning to the risks associated with supply chain vulnerabilities, we are moving towards an environment where organisations will increasingly depend on integrated datasets and AI models at a core operational level. With criminals already taking advantage of AI’s capabilities to improve their effectiveness, the impact of data being manipulated at a supply chain level could be catastrophic.
As a result, business leaders need to plan ahead to ensure they have strong protection and remediation processes in place, including disaster recovery, integrated into their supply chains. The focus should be on prioritising those applications that have fundamental operational importance and are based on what constitutes a minimum viable business and acceptable risk posture. This makes organisations cyber resilient and able to quickly restore systems, data and services in the event of an attack.
Herein lies a crucial point. Implementing AI is already a risk-reward balancing act, and the stakes will continue to rise as the technologies are more deeply integrated into tech infrastructure and business processes. Striking the right balance between innovation and accountability is the only way to unlock AI’s potential without inviting its more dangerous downsides. At the same time, coordinated government-led regulation, however distant that prospect is, remains essential to create enforceable global standards for AI safety and security.
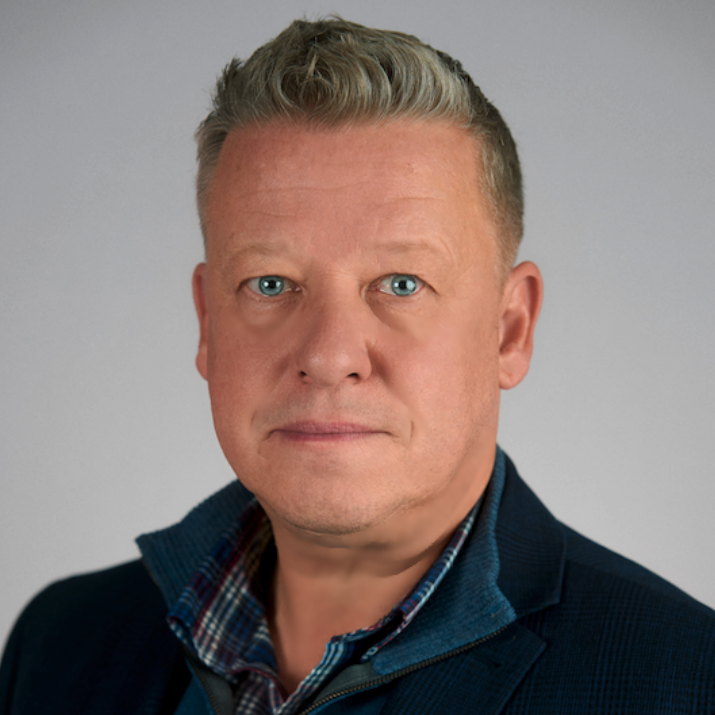
Darren Thomson
Darren Thomson is Field CTO EMEAI at Commvault.