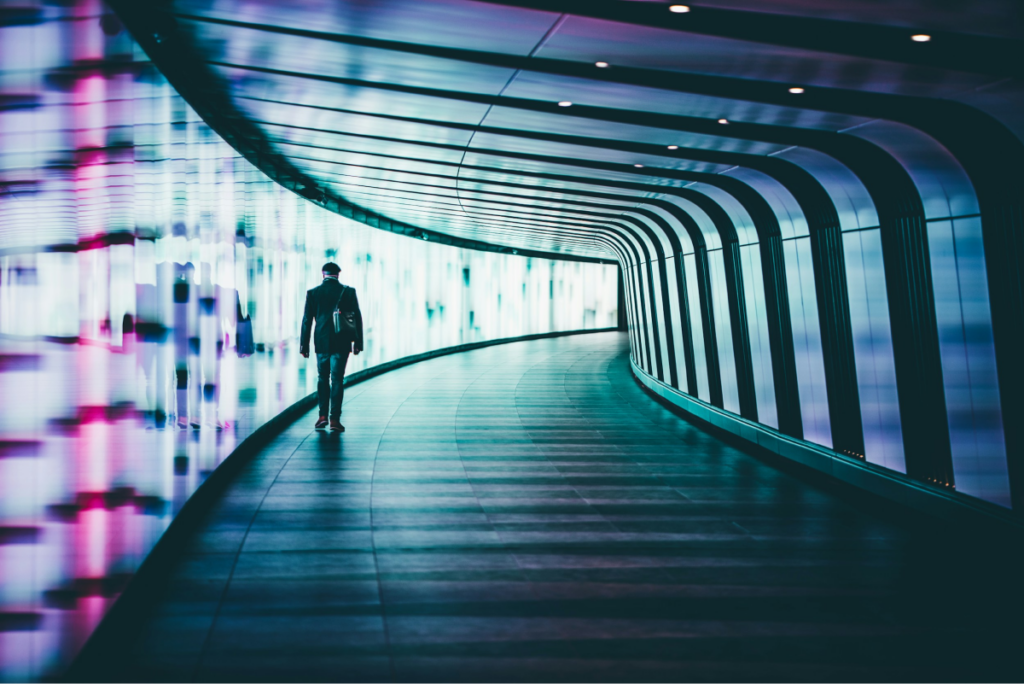
In the latest McKinsey Global Survey on AI, 65 per cent of respondents indicated that their organisations are regularly using generative AI. As businesses increasingly adopt artificial intelligence to enhance their internal operations, it is vital to align AI initiatives with broader business objectives to ensure maximum return on investment.
Data is the linchpin of any AI-led transformation strategy, so businesses must ensure their data infrastructure is ready to provide AI technologies with what they need to accelerate processes, workflows and innovation cycles. Without this foundation, AI-powered applications will not integrate with existing systems.
Further to the existing stack, the impact on employees and day-to-day workflows must be assessed and managed appropriately, as the directionless adoption of AI can lead to a number of risks, such as data loss and shadow AI, where unapproved tools compromise data security. Before jumping into implementing AI-powered technologies, organisations should therefore assess whether AI will address their specific challenges.
Laying the foundations for AI
Before committing to an implementation strategy businesses should evaluate if AI truly suits the specific task at hand. For example, although more than six in ten large companies worldwide are using generative AI, and 74 per cent of those are already seeing sizable returns on investment, this does not mean that GenAI is right for every company, particularly SMBs. In many cases, simple process reengineering can prove more effective than adopting complex AI solutions. For example, deploying a large language model (LLM) for a straightforward use case might be excessive and costly, as operational expenses can quickly rise with a growing user base due to factors like runtime, GPU utilisation, and cloud hosting.
Directionless adoption is also a key concern as it can lead to issues like shadow AI, where employees use unapproved tools to do the job of an approved tool, such as using ChatGPT instead of a Copilot, which then undermines data security and compliance.
Breaking down data silos and enhancing data quality
To fully unlock the transformational potential of AI, a robust data infrastructure is essential. This means that organisations should focus on identifying and eliminating data and process silos before adopting AI to maximise their return on investment. Effective data integration not only sets the stage for successful AI implementation but also ensures that high-quality data is accessible across all departments.
When data is confined within departmental boundaries, it can limit the AI’s effectiveness and hinder insightful decision-making. Thus, breaking down data silos and improving data quality will allow businesses to make the most of their AI investments, as data—the lifeblood of AI—can be operationalised more readily. AI-enabled Enterprise Resource Planning (ERP) systems are a great example of how this can be achieved, as they open up data across systems for use by all departments and teams, while ensuring the right controls are in place in each case. This enables sales, marketing, HR, and accounting teams to harness the same data without the need to manage, store, and secure it on siloed systems.
This holistic approach helps organisations manage and utilise data more effectively while also reducing technology and data management costs. Plus, having all relevant data accessible across different teams encourages collaboration, reduces rework, and empowers teams to make informed business decisions together.
AI integration with existing technology and processes
Evaluating AI initiatives for compatibility with the current technology stack and workflows is important to avoid any unnecessary expenses. Organisations should aim for solutions that effortlessly integrate with existing systems, aligning with long-term goals while also providing quick wins in the short term. This balance helps ensure a smoother transition and maximises the value of investments.
Additionally, organisations can create a roadmap for AI implementation that incorporates behavioural and process frameworks supported by clear policies. This strategy not only mitigates the risks associated with shadow IT but also strengthens data security, paving the way for a more secure and effective AI adoption in the future.
When it comes to working with LLMs and natural language prompts, the human element of any AI initiative should not be overlooked. Businesses can address this through preparing and supporting their employees through behavioural shifts which include, training programmes that improve AI literacy and encourage collaboration between human expertise and AI capabilities. Organisations should also consider establishing an AI advocacy group that provides knowledge sharing and promotes engagement with adopted AI tools to maximise their value.
With the rapid pace of AI advancements, businesses need to foster a culture of continuous learning and adaptation within the workforce to remain competitive. By establishing centralised AI working groups or advocacy teams, teams will be able to remain informed about the latest developments. The same method can be applied to engaging with long-term strategic partners as this way, leaders can keep up to date and access the expertise needed to remain competitive.
Measuring the success of AI tools
Once implemented, there are a number of metrics business can use to determine the success of AI tools. For example, most applications will be able to deliver Monthly Active User (MAU) reports, which can show how many employees are engaging with an AI-powered product or service. If the engagement is low, then training programmes can be delivered to grow the user base and increase engagement and ROI.
Also, user feedback is a useful way of understanding the value-add of any technology, as MAU only tells half the story. If users are engaging with the technology, but are not adding value to their workflows, new educational pathways may be needed.
Looking forward
Ultimately, when it comes to maximising ROI, businesses will benefit the most from starting small and focusing on building a solid data infrastructure that eliminates silos and unlocks the use of high-quality data across the organisation. Silos don’t just relate to data, they determine bespoke processes and establish separate ecosystems whereby departments drift further from the organisation’s core infrastructure and processes.
To become truly AI-ready, data infrastructure, behaviours and processes must align. This is the ideal foundation from which businesses will be able to seamlessly onboard new solutions and capabilities as they come online, ensuring that they benefit from the rapid AI-led innovation occurring across industries.
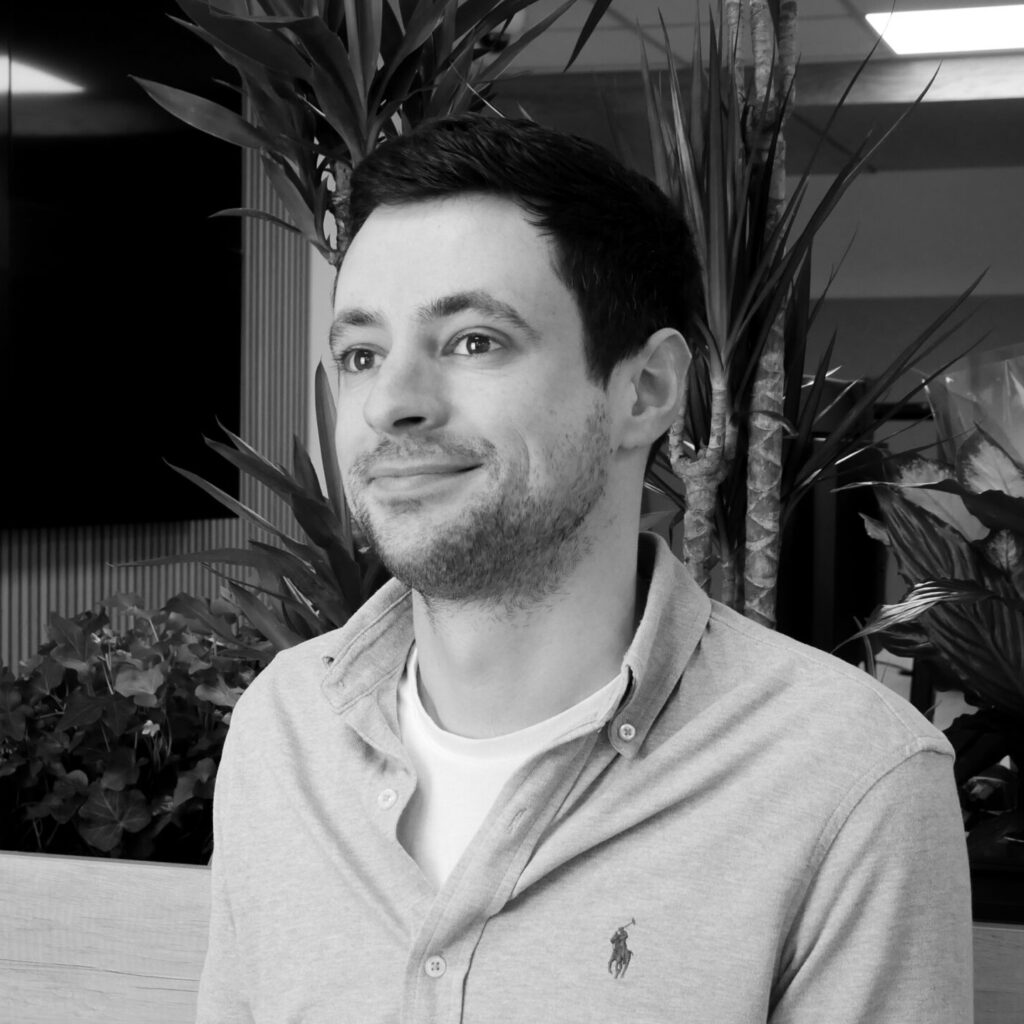
Tristan Shortland
As Chief Innovation Officer, Tristan leads Infinity Group’s Innovation Lab and is responsible for driving new business, providing presales architecture and consultancy, and product development.