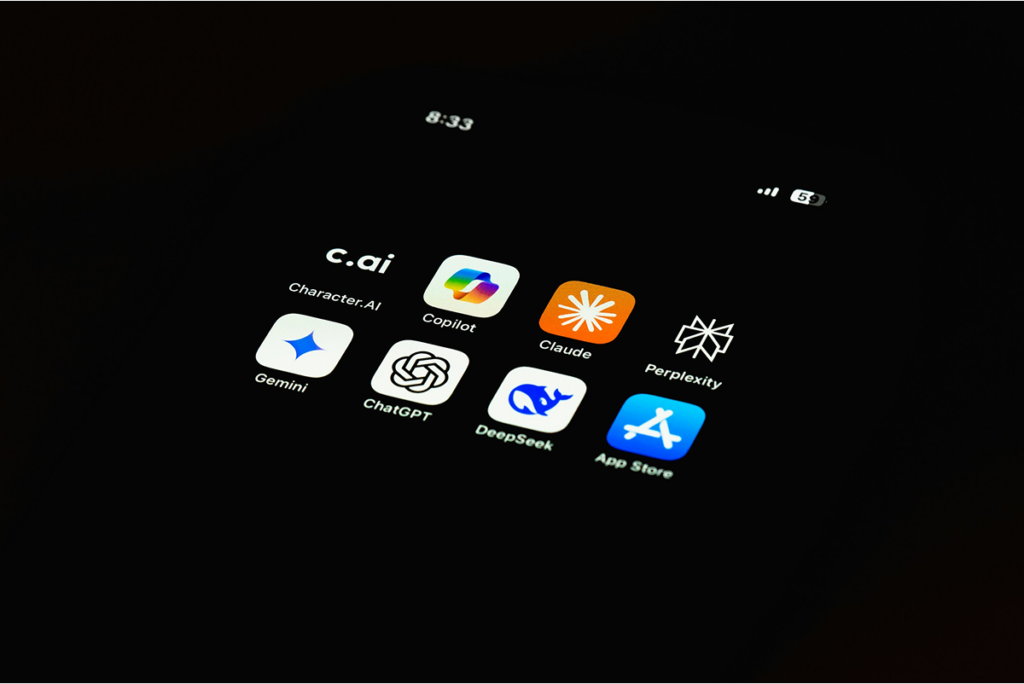
The announcement of the DeepSeek R1 model in January by a private Chinese company shook the AI industry to its core. DeepSeek R1 seemed to provide equivalent capabilities to ChatGPT, while costing far less to create. DeepSeek’s launch caused $1 trillion tech market loss and has been described as a “wake up call” by Donald Trump, and a “Sputnik moment” by leading industry commentators like Marc Andreessen.
DeepSeek says it has been able to train its model at a cost of only $6m (£4.8m), which is a fraction of the overall $100m alluded to by OpenAI when training GPT-4. It has also seemingly been able to minimise the impact of US restrictions on the most powerful chips reaching China.
DeepSeek’s founder reportedly built up a store of Nvidia A100 chips, which were banned from export to China since September 2022. He seemed to pair these chips with cheaper, less sophisticated ones – and in so doing, chanced across a much more efficient process.
So, what exactly is DeepSeek and why did its announcement cause such interest and market disruption?
1. Necessity is the mother of invention
For various geopolitical reasons the US has decided to enforce export restrictions that have targeted China’s ability to make advanced semiconductors, and banned the export of high performance chips of the kind (e.g. Nvidia) that are currently enabling US companies to scale up their models.
US AI companies such as OpenAI, Google, Microsoft and Meta have therefore been able to feast on increased chip capability and power consumption to scale up their models and boost their capabilities. This approach has led to concerns regarding the Big Tech need for additional power – Microsoft for example has brokered a deal to reopen the Three Mile Island nuclear plant (planned for 2028) to power the burgeoning power demand for its models.
China on the other hand, has been starved of high AI performance chips and this has forced emerging Chinese AI companies like DeepSeek to adopt approaches that minimise power consumption. Ironically, for the US policy makers this has made the DeepSeek Chinese model more and not less capable, owing to the innovative methods used to train the model on less power.
2. Bigger isn’t necessarily better – it’s what you do with it
It turns out that whilst size matters in the world of AI, it isn’t the only factor that drives performance. Various advanced techniques can help to train models without the need for massive chip computation or power consumption. The unusual thing about the DeepSeek model training is that although these advanced training and inference methods were well known, they hadn’t been used in conjunction with each other. By openly publishing their approach, it became clear that the surprising innovation was that DeepSeek had been able to harness the power of a number of these separate techniques together in symphony to achieve exceptional performance against industry benchmarks.
The techniques used by DeepSeek included:
a) Mixture of Experts (MoE) – a technique that enhances efficiency dynamically activating only a subset of specialised “expert” subnetworks for each input. Unlike traditional dense models, which process all inputs using the entire network, MoE routes different parts of data to different experts, significantly improving computational efficiency while maintaining high performance.
At its core, a MoE model consists of:
- Experts: Independent neural network modules, each trained to specialise in different aspects of data.
- Guides and gates: A trainable component that learns to select the most relevant experts for a given input.
By activating only a fraction of the total model parameters during inference, MoE enables LLMs to achieve superior performance with reduced latency and energy consumption.
MoE is rapidly shaping the future of AI by enabling larger, more intelligent models without proportional increases in computational demand.
b) Knowledge Distillation (KD) – a technique where a smaller, more efficient student model is trained to replicate the behaviour of a larger, more powerful teacher model. This process allows AI models to retain high performance while reducing computational costs, making them more practical for deployment.
DeepSeek used distillation to refine its large-scale models, ensuring that efficiency does not come at the expense of intelligence. By transferring knowledge from a larger model, DeepSeek used lightweight versions while running efficiently on consumer hardware, and allowed student models to learn implicit patterns from the teacher, improving their ability to handle diverse tasks with fewer parameters.
This all leads to lower computational costs, since a distilled model requires fewer resources, making AI deployment more sustainable and cost-effective.
c) Chain of Thought (CoT) reasoning – a technique that enhances AI’s ability to solve complex problems by breaking them down into step-by-step logical reasoning. Instead of providing direct answers, the model articulates its thought process, leading to more accurate and interpretable results.
Daniel Kahnmann wrote a famous book “Thinking Fast and Slow” that described the human ability we have to think either very quickly and instinctively (System 1 – and this is like typical LLM generations), or think in a more step-wise, slower and structured way (which he called System 2 – e.g. performing long division, or breaking down a complex problem)
CoT can be likened to forcing an LLM to use “System 2” type thinking to improve:
- Mathematical and logical reasoning, crucial for structured problem-solving.
- Complex decision-making, by following a structured reasoning path.
- Transparency, making AI outputs easier to verify and trust.
By integrating CoT, DeepSeek models demonstrate better performance in problem-solving abilities, improving performance in maths, coding, and multi-step reasoning tasks.
3. Opening up the AI market
The final and perhaps most remarkable point about the DeepSeek announcement was that in an AI revolution “wild west“, that is increasingly aware of the value of AI, it has become unusual for commercial AI to fully open source their models, code and training approaches. Being fully “Open” means that anyone can use the models and code, and understand how the training was undertaken to further fine tune the models.
For example, Elon Musk has famously sued OpenAI for not being “open” – he claims that the organisation he helped launch to freely advance AI for humanity has now become a profit-driven powerhouse. OpenAI, Google, Microsoft, Anthropic, and most of the US tech AI powerhouses, have not made their models Open (Meta is the only notable example of an organisation who has truly aligned with “Open” principles).
There seems to be some growing evidence that DeepSeek has somehow ‘ripped off’ ChatGPT
DeepSeek on the other hand, was completely transparent about publishing its model weights and training approach. This converted industry sceptics very rapidly to believers, as they realised that the model performance (so often obfuscated and difficult to understand or replicate by US tech firms) could be actually tested and replicated. Not only that, but since the models were open they could be run, modified and verified on independent infrastructure (e.g. hosted in the EU or US), and not just via the DeepSeek API endpoints on Chinese infrastructure. Indeed, Microsoft took the unprecedented step of immediately launching the DeepSeek model as part of its Azure AI Foundry. Perhaps Microsoft and OpenAI are not in as cosy a relationship as they seem.
4. …or ripping it off?
Whilst DeepSeek’s adherence to “open” principles might seem on the face of it to be admirable, some industry analysts have questioned the veracity of their approach, and there seems to be some growing evidence that DeepSeek has somehow ‘ripped off’ ChatGPT. The output of DeepSeek and ChatGPT are strikingly similar while other models (e.g. Gemini, Grok, Anthropic) produce very different outputs. DeepSeek also seems surprisingly “Westernised” in its responses for a Chinese model (except for certain seemingly censored information regarding Tiananmen Square..!). It assumes American food, programming templates, sports preferences, entertainment – strange for a Chinese model to be so American in nature. Finally, there are numerous examples of responses where DeepSeek believes that it is ChatGPT which could be proof – a smoking gun – that, DeepSeek was indeed trained on OpenAI models.
Conclusion
There can be no doubt that the DeepSeek announcement was more than just an AI industry announcement, but a huge geopolitical statement to the world that China is not as far behind the US as was previously assumed (at least in terms of AI development). The fact that the announcement was made around the time of the Trump inauguration was surely no coincidence.
The announcement of DeepSeek caused the largest ever stock market crash in a single day (over $1 trillion dollars), affecting pension funds and investors worldwide, and this has again underscored the importance and emerging relevance of the AI revolution to the world.
But aside from politics, the announcement was also laced with poignancy regarding the nature of innovation – David can beat Goliath by the application of innovative techniques and being forced to think in a different way (in this case because of chip paucity).
This also has important and beneficial implications in terms of energy consumption – it may not necessarily be true that more powerful models need more power. Our brains for example run on 20W of power – which is equivalent to the lowest power lightbulb.
Finally – as a neuroscientist I also find this DeepSeek announcement particularly fascinating since it demonstrates AI more closely approximating models of neuroscience. Building on the attention mechanisms (that are key to the Transformer architecture) that crudely approximate to a model of perception and consciousness, the Mixture of Experts (MoE) model is similar to how the brain operates with specialised brain centres. We have specialised areas to deal with our modalities – such as speech, vision, hearing, touch, and the MoE / distillation techniques approximate to this. Similarly the Chain of Thought reasoning and “System 2” thinking approximate to the key functions on the pre-frontal cortex that force a slower elaboration of thought, world view, and planning.
I am excited to see how further developments in AI will mirror neuroscience further to achieve the remarkable power efficiencies achieved by our brains. Firing patterns of neurons in the brain are critical to efficient neuronal function and not currently well modelled. As an example. “Liquid neural networks” are a fascinating area of frontier AI research where, inspired by human neurons, extremely adaptive models can be run on extremely lower power, and yet no one has (yet) harnessed this commercially. I’m sure there are many more DeepSeek-like deep shocks to come.
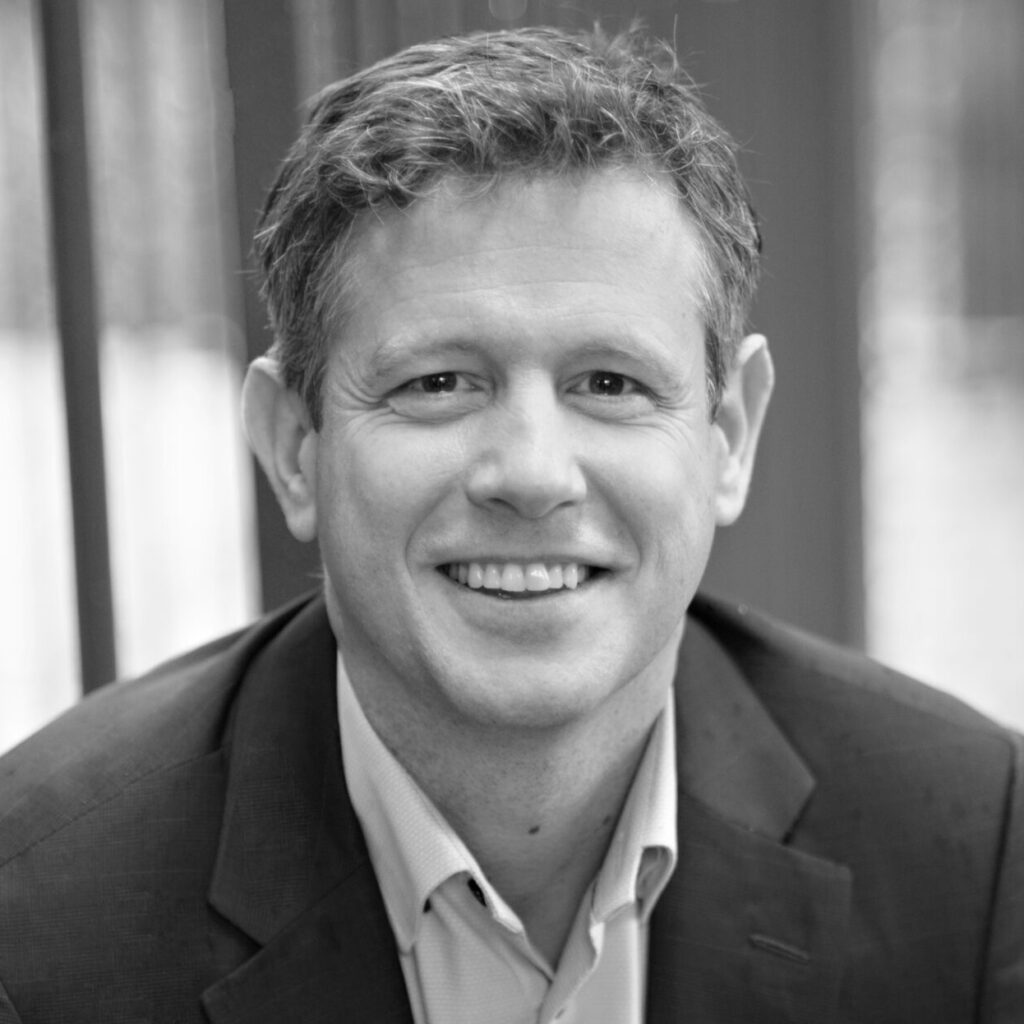
Steve Elcock
Steve Elcock is a Neuroscientist and Product Director – AI and HCM at Zellis.