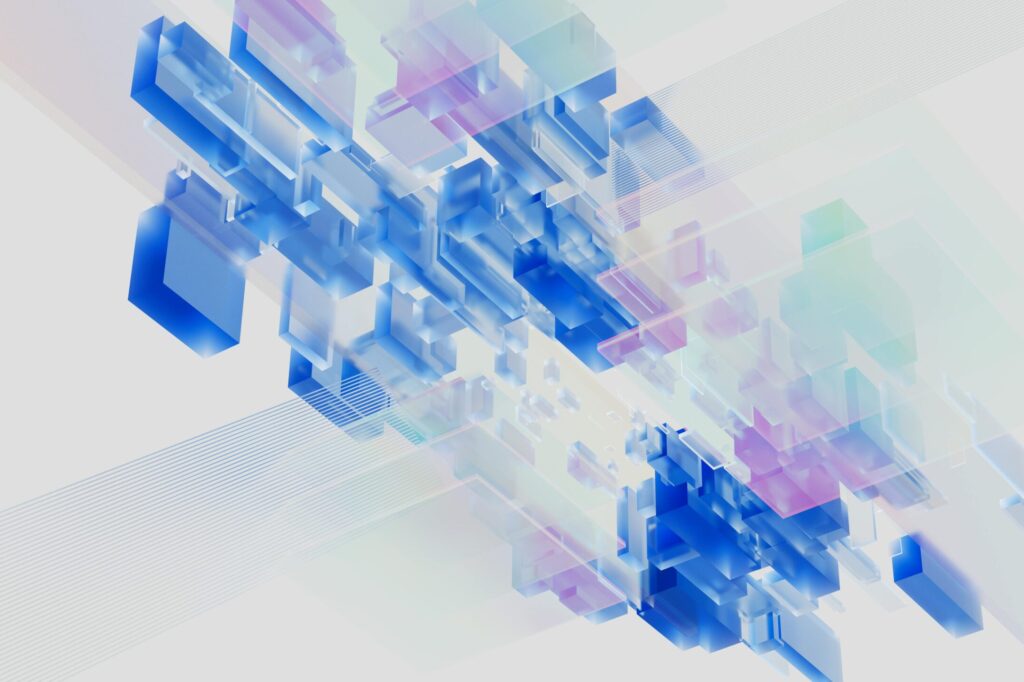
With organisations of every shape and size rushing to implement AI tools and services, as well as develop their own AI-powered solutions in recent years, a key concern among business leaders and decision makers is the level of ROI they can expect from AI projects.
The automation of workflows and manual processes is the typical draw for most AI projects. Depending on the use case, the level of complexity for these projects varies greatly, and with greater complexity comes greater investment of both money and time. As with any technology implementation or transformation programme, there has to be a realistic timeframe and qualifiable KPIs that set out the solution’s value. On the operations side, the result is a practical solution that delivers value for the business. For the team in charge of delivery, it’s a case of justifying the timeline and showing that the project will meet its objectives.
Of course, it is possible that an AI project might fail, but there are a number of tips and tricks that will ensure that, at the very least, the time and effort is not spent in vain, and at most will result in the delivery of a transformative solution. With this in mind, here is some advice to ensure that AI projects deliver maximum value.
Well defined project proposal
The most common reason AI projects fail is when there is a lack of a solid objective. When the aims are not well defined, the project and its assigned teams will lack focus and unravel. This is the earliest sign that a project is not viable, however, it’s also early enough in the process to refocus and establish a more specific end goal.
A project plan should include a clear objective and well-defined success criteria. It’s essential to scope out the pain point and determine whether using AI is the right approach, or if there’s a simpler way to solve the problem. From there you need to have the right level of investment in place to secure the appropriate development and resource bandwidth, as well as ensuring relevant data can be accessed as needed. Legal and governance clearance for the project is also key, particularly for projects in regulated industries like finance and healthcare.
To get the most out of advanced technology, it’s crucial to push the boundaries and limits of your technical teams, as this is where transformative innovation can occur.
Quite often, AI projects are put on hold as data scientists will need to wait for the use of certain datasets to be authorised. Other times, we just need to wait for higher quality datasets to become available. These are two of the biggest issues, but are usually resolved over time.
In my experience, AI projects are rarely dropped entirely, as there are always elements of the work that can be reused elsewhere to cut down rework.
Ensuring the right amount of friction
Much like a team principal in F1, the CISO will make the important decisions to ensure the correct amount of friction is applied to enable employees to work securely. Too much friction and the employee will struggle to keep up with their daily tasks and complain of a poor or frustrating experience. Their eyes will be diverted to watch other teams who can perform faster with less friction. Not enough friction and it’s likely they will encounter an incident (/tyre wall) that ultimately ruins everyone’s weekend. So how do you manage this?
The answer is through continuous assessment of these variables. For example, tools like a modern SASE (Secure Access Service Edge) platform combine analytics telemetry, much like that on an F1 team’s pit wall. This information can not only be presented to the employee but also to the network and security operations team and CISO. Outlier variables impacting performance or security can be discovered early and mitigated before they become a bigger problem, and policies and routing can be adjusted to maintain optimal performance. This is done through dynamic policies that determine the composite score of these risk variables and set actions (Allow, Block, Alert, Coach/Educate, Redirect).
Measuring success
When you’re very specific about the end goal from the outset and have clearly defined the problem you’re trying to solve, it’s much easier to measure success. While it’s tempting to aim for 100% performance, that’s not always realistic. If your existing manual process is only delivering 20%, and your new model takes you to 70%, then you’ll have met a success criterion of delivering an improvement.
It’s also important that data science teams are testing themselves against what’s possible with the technology, as this approach can deliver breakthrough solutions that may exceed expectations. When projects don’t quite fully deliver the desired solution, it’s essential to debrief and share learnings. Determining what could have been done better and approaching the next project equipped with this knowledge is a measure of success that should not be underestimated.
Don’t fear failure
Many organisations today have internal innovation labs, or at least some allowance for experimentation with new technologies. This should be the case with any business experimenting with AI. To get the most out of advanced technology, it’s crucial to push the boundaries and limits of your technical teams, as this is where transformative innovation can occur.
The important thing with any AI project, however, is to fail fast—which usually means putting the project on hold until certain requirements are met, such as data access, legal clearance or the availability of a new tool. In other instances, exploring potential technical oversights might be what’s needed. Are there system defects? Has model performance degraded since deployment? If so, teams need to prioritise and plan for continued model maintenance over its lifecycle
For data scientists, projects may not always be successful, but as they explore a new technology, they may identify new learnings that will serve them and their teams well into the future. With research and development in AI increasing by the day, it’s always better to pause a project that may not be on track, rather than abandon it entirely. New capabilities and techniques are emerging all the time, which unlock new possibilities and use cases.
Four years ago, a lot of natural language processing tasks that we take for granted today would not have been possible. This is due to the explosion and availability of LLMs. With the level of research and development that is going on in AI, it’s likely that a solution that solves a problem with a paused project may become available in the near future. My advice, therefore, is to first establish a balance between ambitious and well-defined objectives and then be bold in how you achieve them.
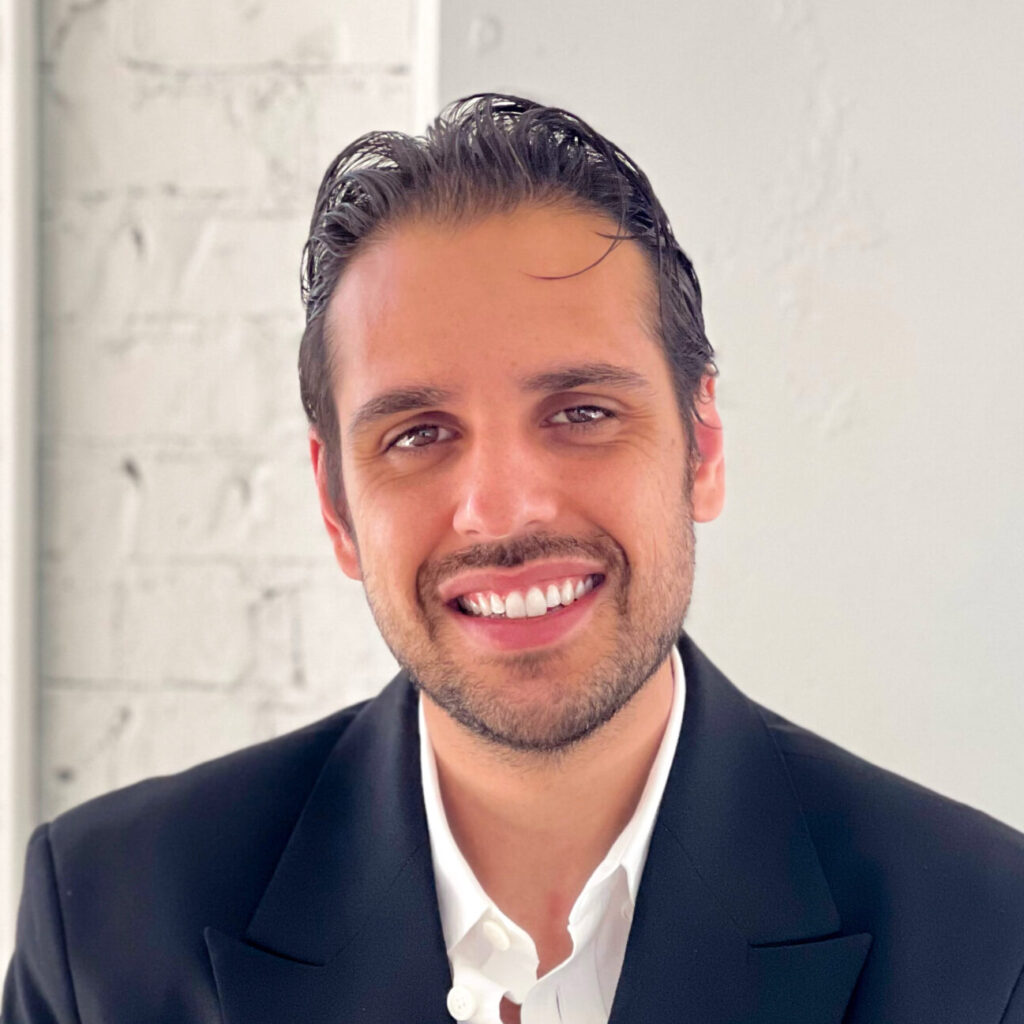